Final report for GNC18-264
Project Information
Increasing agricultural production while also preserving biodiversity and ecosystem services is difficult and likely only possible with an understanding of the spatial variation of soil communities as pertains to soil health. A spatial biological indicator of soil biodiversity could provide an important link between soil condition, biodiversity, and resultant ecosystem services, but such an indicator is currently lacking. Soil microarthropods such as springtails (Collembola) are extremely common and dense in the upper soil (up to hundreds of thousands per square meter) and are as much a part of the soil as they are in contact with it. They are therefore likely to serve as an excellent biological indicator of soil conditions. This project will sample the Collembola communities in several land use types, including two types of agriculture: "conventional" and "agroecological." The composition of Collembolan communities will be used to quantify the link between these arthropods and indicators of soil nutrition, including carbon and nitrogen pools, with the aim of developing a Collembolan-based biotic index of soil health. The spatial variation in soil conditions and in Collembola communities will be mapped within sampled fields and made available to participating farmers. In doing so, this project will lay the basic groundwork for future applications of a bioindicator and a biological liaison of soil health.
The project ultimately aims to develop an efficacious biotic index based on springtail communities. Similar to pollution indices based on aquatic invertebrates, the presence or absence of Collembola, certain genera therein, or how many there are (abundance) should be able to rapidly indicate the overall nutritional state of the environment (in this case, the top soil). Additionally, we intend to cross-validate such an indicator with spatial statistics, in order to assess how well-matched soil conditions are to Collembolan community metrics over space and time. With this being of necessary concern and interest to farmers, we plan to continue engaging Indiana farmers for the development of such an index, at no expense to the farmers other than in allowing us to sample from their fields. In the process, we hope to not only inform involved farmers of the state of both their soils and soil biodiversity, but we will also:
1.) Use this as an opportunity to educate about cryptic soil biodiversity, with Collembola as our model arthropod, garnering interest in the prospective bioindicator by recruiting further collaboration for the project.
2.) Continue the tradition of both NCR-SARE and Purdue Extension in developing and encouraging sustainable and innovative methods of agricultural monitoring among local farmers.
Research
Field study sites for the overall dissertation project fall under five different land-use categories located within Indiana, chosen both for their assumed ability to best represent the dominant landscapes that can be encountered in the state’s geopolitical boundaries, and on a gradient of increasing land-management "intensity:" secondary-growth forest, clearcuts or successional forest shrub, restored native prairie, agroecological farmland, and conventional farmland. Each of these land-use categories were surveyed with three transects to generate a representative picture of Collembolan communities and soil dynamics within each, for a total of 15 transects per sampling year. However, due to SARE's interest in agricultural pursuits, the grant provided funding only for the two agricultural landscapes (which constituted 6 of the 15 transects).
It was decided that a conventional farmland or field was defined as those under monocultural crop production, utilizing conventional pest management strategies, and, for ease of comparison, all fields in this category grew either corn, soy, or a corn-soy rotation. An agroecological farm was qualified as being either USDA-certified organic or otherwise employing alternative agricultural techniques or practices associated with "sustainable" or "traditional" agricultural production, such as utilizing permaculture-type practices or cultivating diverse, polycultural vegetable crops. Six different fields across five different farms (with two of the fields being under the same management) were surveyed in this study, with each field containing one of the three transects per either the agroecological or conventional category. All of these are located in counties across northern Indiana. The agroecological farms included in the study are, briefly, as follows: an USDA-certified organic corn-soy monoculture (coded as SHO in the data), and two, community-supported (CSA) polycultural farms, Perkins’ Good Earth Farm (coded PC) and Lanes’ End Farm (coded LAN), respectively, either of which grow a variety of specialty or heirloom vegetable and fruit varieties. SHO is under a no-till/strip-till regimen (no-till: 2017, ridge-till: 2019), while both PC and LAN made use of no-till, mulch composting, and/or turning under green manure for various vegetable plots. The conventional cropland included two monocultural fields under a corn-soy rotation (SHC and WRN), and one monocultural field of continuous soy (coded LNG), all of which made use of conventional tillage practices. All of the farms are located in counties across northwestern Indiana.
Two sampling years have so far been conducted, with the first year occurring in the summer of 2017 and the second in the summer of 2019. The second sampling year (2019) was limited to only the two agricultural land-use sites, as per the stipulations of the grant. Both years made use of the same transect sampling method, utilizing a novel grid-like transect in the form of a partial-Fibonacci spiral (Figure 1). This spiral conformation was selected as being best suited for the spatial component of our project, given that it eliminates directional bias, maintains good coverage, and maximizes intersample distance variation (Fortin and Dale, 2005). Each spiral had 20 sampling points, ranging approximately 80 meters in diameter at the furthest points of the spiral. This distant was selected for as ideal both for the golden ratio calculations placing the five core points of the spiral at distances similar to those found on typical grid or line transect sampling for Collembola (approximately 3 to 5 meters apart), while also not exceeding the areas of our smallest landscape units (our small-scale production polycultural farms). All of our transects were randomly placed within the research areas by selecting random coordinates in R.
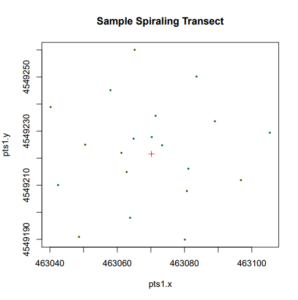
At each point of the transects, one large soil core (approximately 13 cm wide x 20 cm deep) and two to three smaller cores (3 cm wide by 20 cm deep) were taken within an approximately one meter-squared area, centering around the large core. The smaller cores were subsequently pooled to average the soil properties within the given area. This resulted in a total of 120 large cores and 120 small cores (after pooling) collected during either sampling year for our agricultural land-use sites, totaling 240 large and 240 small cores for both years of sampling. The larger core is dedicated to the extraction of Collembola, either via a sugar flotation procedure modified from Snider and Snider (1997) following soil-washing as for the 2017 sample collection, or, as for the 2019 samples, using a Tullgren funnel extraction method. A morphological identification system was decided upon for Collembolan identification, first to the superfamily level and then to the tribe or genus, if necessary, to obtain good taxonomic resolution of the sampled areas while avoiding time-consuming species-level identifications.
The pooled, smaller cores were devoted to chemical and physical analyses of the soil properties. Chemical measurements to determine some of the most pertinent physical and organic properties of the soil included: percent soil moisture content (taken on site); pH; soil texture; labile carbon (POXC using infrared spectroscopy); and an inorganic nitrogen measurement (using a SEAL analytic machine). Taken together, the data obtained on these soil properties and Collembolan community metrics is intended to be used quantify any relationships that exist between them using nonlinear regression and redundancy analysis, whereas an asymmetric bioindicator procedure (IndVal) will then be used to examine the specificity and fidelity of the biota to these conditions. Additionally, variography and kriging will be used via R and QGIS to examine the spatial extent over which both the Collembolans and the soil conditions vary, allowing us to project how Collembolan communities change across space within these landscapes, and how well matched the biota and conditions are to assess how suitable the biotic index is for describing both intra- and inter-site variation across our different land-use categories.
To date, all 120 of the Collembola cores collected from the 2017 sampling season have been washed, floated via sugar flotation, and sorted to superfamily/family. The following taxa were represented in the data: Onychiroidea, Entomobryidae, Isotomidae, Sminthuridae, and Hypogastruridae. Another category of "unidentified" was used to indicate Collembolans which could be identified as such but which were too damaged to initially determine their family under a dissection microscope. Two replicates per each of the 120 sample points were run for the inorganic nitrogen extraction assay, as well as two replicates per sample point for labile carbon (POXC) analysis. The 120 Collembolan cores collected for the second sampling year in 2019 were extracted via Tulgren funnel and have been similarly sorted to the superfamily/family level, though the data as well as the chemical assays remain to be appropriately compiled for analysis and comparison to the first sample season.
Only two out of 120 data points in the 2017 data set returned no Collembola specimen, and so these were excluded from our analyses. A Welch's one-tailed t-test was first performed for both Collembolan diversity (calculated using the Shannon diversity index and with the exclusion of Sminthuridae due to low representation across all transects) and abundance, comparing the agroecological specimens against those found in the conventional fields. While diversity was non-significant (t=0.91486, df = 115.78, p-value = 0.1811), the t-test for abundance between the two landscape types revealed a significant difference (t = 2.6404, df = 65.406, p-value = 0.01034), with the average abundance in the agroecological category (65.78) being higher than that found from the conventional sites (26.33). We suspect a significant difference in diversity was not noted because of our preliminary sorting being situated at such a high/unrefined taxonomic level (family/superfamily), and that differences in the communities in this respect may emerge once genus-level sorting is completed. When represented visually, as in Figures 2 and 3 below, we can see that all of the agroecological sites (coded as LAN, PC, and SHO) have higher total abundances than two of the three conventional sites (coded LNG and SHC).
As for the remaining conventional site (WRN), it demonstrated a higher or similar average abundance to two of the three agroecological sites (LAN and SHO), and, on its own, accounted for most of the total abundance for the conventional sites, given that both LNG and SHC often had few Collembolans per sampling point (Figure 4). Similarly, one of the agroecological farm sites (coded PC) contributed to most of the total abundance for the agroecological land-use category, also shown in Figure 4.
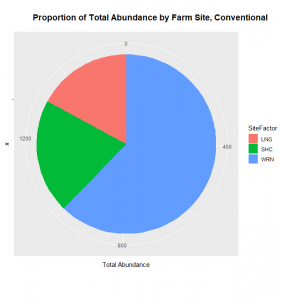
How these the individual sites differed from each other was explored further in the context of the physio-chemical parameters thence far compiled — namely, nitrite/nitrate concentration (mg N/L), ammonia concentration (mg N/L), labile carbon (mg/Kg), and soil moisture percentage. Of these, only nitrite-nitrate concentration was statistically insignificant between the two farm types when compared via t-test (t = 1.4634, df = 68.977, p-value = 0.07395): the other three dynamics were significantly different between the agricultural types (ammonia concentration: t = 3.0975, df = 86.087, p-value = 0.001317; labile carbon concentration: t = 5.5852, df = 68.192, p-value = 2.206e-07; and soil moisture percentage: t = 3.9404, df = 115.28, p-value = 6.993e-05), with the agroecological type demonstrating consistently higher average concentrations/percentages than the conventional category. A comparison of means by individual site and farm type is represented visually in figure 4. These findings seem to be consistent to what might be expected from precedent in the the literature: with greater ground cover by vegetation due to the tolerance of weeds and/or grasses in the agroecological fields, and with the use of green manures, cover cropping, and/or compost to maintain fertility, the agroecological fields generally retained moisture better, had a higher concentration of ammonia from decaying/humifying matter, and had a greater concentration of labile carbon, possibly indicative of a more stable soil carbon pool than what is typically found under conventional cultivation (Leifeld et al., 2002; Fließbach et al., 2007; Martyniuk et al., 2016; Liu et al., 2018).
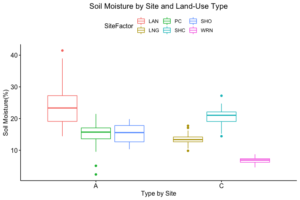
The general exception to this pattern across the variables was consistently the transect/field in the agroecological category coded as SHO. SHO had concentrations of nitrite/nitrate, ammonia, and labile carbon more similar to the conventional sites than to the other two agroecological sites (PC and LAN), the latter of which it was consistently lower than. SHO similarly had a total Collembolan abundance more similar to the conventional sites (Figure 2) than to the other transects in the agroecological category. As indicated in the Materials and Methods section, SHO was an USDA-certified organic corn/soy monoculture, under soy cultivation at the time of the 2017 sampling, whereas both PC and LAN were small-scale polycultural farms growing a variety of heirloom vegetables for community-supported sale. We suspect, as such, that the monocultural form of cultivation itself was the most influential in determining the abundances of Collembola and markers of soil fertility like inorganic nitrogen and soil carbon — but we are as of yet unable to determine this statistically, due to the relatively small sample size.
To determine which of the soil variables had the greatest effect on Collembolan abundance, both as a total and at individual taxonomic categories, ANOVAs were run on each of the four physio-chemical properties as independent variables in respect to total Collembolan abundance. A stepwise regression was then run including these variables as well as the categorical factors of farm type and individual transect to select the most parsimonious and explanatory model for total abundance via Aikike's Information Criteria. Table 1 shows the ANOVA results for the four soil variables. As can be seen, neither nitrite/nitrate concentration nor soil moisture concentration are significant predictors of total Collembolan abundance when considered on their own — but when the two-level factor of farm type is included in the analysis, the models then become significant (nitrite/nitrate + Farm Type: f = 3.931 on 2 and 116 DF, p = 0.022; soil moisture percentage + Farm Type: f =4.78 on 2 and 116 DF, p = 0.01). When nitrite/nitrate and soil moisture percentage are considered against the abundances of the four major superfamily/family groupings irrespective of farm type, the only significant figure (f = 7.487 on 1 and 117 DF, p = 0.007) is for the influence of soil moisture on the abundance of Entomobryidae, an epidaphic/surface-dwelling family of springtails. This is likely a result of the greatest amount of Entomobryidae being collected from one of the agroecological transects, LAN, which, as displayed in Figure 4, also had the most saturated soil of all the farms sampled. When farm type is considered in the analyses, nitrite/nitrate concentration also becomes a significant predictor (f = 6.155 on 2 and 116 DF, p = 0.003) of Entomobryidae abundance, while soil moisture percentage also becomes a significant predictor for the abundance of the superfamily Onychiuroidea (f = 6.155 on 2 and 116 DF, p = 0.008) as well as for Entomobryidae (f = 6.943 on 2 and 116 DF, p = 0.001).

Both labile carbon concentration (denoted as POXC in table 1) and ammonia concentration, when considered as the only independent variable, act as significant predictors for total abundance. When compared to individual family abundances, ammonia concentration is only a significant predictor when compared against Entomobryidae abundance, both alone (F = 11.77 on 1 and 117 DF, p-value = 0.0008) and when further constrained by agricultural type (F = 9.149 on 2 and 116 DF, p = 0.0002). Conversely, POXC was not a significant predictor of Entomobryidae abundance save when the agricultural type was also considered in the model (F = 5.573 on 2 and 116 DF, p = 0.005), but it was a significant predictor for the other three taxa (Onychiuroidea, Isotomidae, and Hypogastruridae), both when considered as the only independent variable and when regressed with the agricultural factor.
Between all of the independent variables, the stepwise regression implicated that the optimized model consisted of total abundance as dependent upon the effects of labile carbon concentration and soil moisture percentage (f=11.34 on 2 and 116 DF, p=3.182e-05). This reflects on how, when the abundance is partitioned into different Collembolan taxa, soil moisture was the variable that was the most related to Entomobryidae abundance, which in turn was one of the chief taxa driving the variation between the two farm types, whereas labile carbon concentration seemed to be aligned with the higher abundances of the other three taxa. It is worth noting that the effect of soil moisture on Entomobryidae abundance as detailed above is a surprising result, given that many species within the Entomobryidae, as epidaphic organisms, tend to be better-equipped physiologically to protect against dessication compared to more-euedaphic (soil-dwelling) species of Collembolans (Chistiansen, 1964; Vegter et al., 1988). One possible explanation for this is that both soil moisture and the abundance of Entomobryidae are simultaneously influenced by a variable that was not here quantified: for instance, as the bulk of Entomobryid specimens were collected from a single agroecological site (LAN), features particular to this location — such as the diversity of surface vegetation in the polycultural rotation, the minimization of exposed soil by maintaining grass-covered lanes between plots, heavily-planted margins, etc. — could be responsible for both the abundance of Entomobryidae and a soil that can maintain higher saturation. This, however, is speculative without data that could not be incorporated into the present study due to logistical constraints (such as a metric for vegetation coverage and/or diversity).
In terms of the spatial structure of the Collembolan communities, semivariograms were run for each of the six individual transects to examine spatial autocorrelation in terms of total abundance, individual superfamily/family abundances, and the four physiochemical variables, and kriging maps were further derived from these results to better visualise how the taxonomic and physiochemical dynamics were distributed within the transect area. It was found that, with few exceptions, many of the abundances and the physiochemical properties were often spatially-unstructured, exhibiting a "nugget effect" as such. This can happen when sample size is not sufficiently large and/or at an appropriate scale to capture any autocorrelation that exists, or if, rather than exhibiting a relationship where points that are closer together are more similar as expected, the inverse is instead found (the further points are more similar to each other than points that are nearer in proximity) for certain topographical/physical peculiarities.
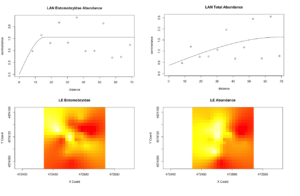
However, from what has been analyzed thence far, the agroecological category exhibits slightly more structure in Collembolan abundance and the physiochemical properties than the conventional category, possibly indicative of a greater stochasticity introduced to soil communities and biogeochemical cycles by the disturbances associated with conventional cultivation. One of the agroecological farms, LAN (Lane's End), had the most-structured Collembolan communities (Figure 5), with the three most abundant taxa that constituted most of the by-catch (Onychiuroidea, Istomoidae, and Entomobryidae) all demonstrating a spherical to linear relationship. Three of the four soil variables (labile carbon, nitrite/nitrate, and ammonia) likewise demonstrated spherical and linear dependency. This and some of the patterns that emerged in the agroecological site PC are suggestive that the scale on which sampling was done was generally-appropriate for capturing autocorrelation in these particular variables, but the four other sites included in the study (SHO, SHC, LNG, and WRN) may simply be too spatially-stochastic in terms of Collembola distribution and the soil dynamics examined to have been detected on the same scale. All four of these transects were situated in monocultures, and so this stochasticity might be related to monocultural cultivation, though this extrapolation requires further exploration with more data in future studies.
To summarize the findings to date: data from the agroecological farms involved in the study suggest a greater ability to foster Collembolan abundance, as well as demonstrating higher ammonia concentration, a greater amount of labile/organic carbon, and a higher soil moisture retention. The agroecological field that demonstrated the lowest values and which was more similar to the conventional type in most of these aspects was an organic monoculture, as opposed to the other two agroecological transects which were situated on CSA polycultural farms. We suspect that the monocultural cultivation itself, irrespective of organic amendments, explains this discrepancy, but the recruitment of more farms would be needed to satisfactorily explore this question. Of the soil variables measured, labile carbon concentration and soil moisture were the most predictive of total Collembolan abundance. In terms of the spatial structure of the communities, the agroecological sites of LAN and PC demonstrated the clearest degree of spatial autocorrelation, whereas the organic monoculture (SHO) and the conventional sites (LNG, WRN, and SHC) often exhibited nugget effects in the semivariance of the soil dynamics and the Collembolan abundance, both as a total and when considered by individual families. The second year of data (2019) is currently being processed and compiled, and will help elucidate whether some of the speculated patterns noted here are a consistent phenomena
Educational & Outreach Activities
Participation Summary:
Due to the ongoing processing and compilation of data for the project, journal publications and Purdue Extension press releases or documents are still pending. One brief, on-site talk was given to interns during a visit to one of the agroecological farm sites participating in the project (Perkins' Good Earth Farm) in the summer of 2019, however, in which the project's purpose, its details, and its desired outcomes were discussed. A manuscript based on findings/results for the 2017 sampling year is currently in revision, and will be submitted by the end of February 2021.
Project Outcomes
There has been interest expressed in the literature and by policymakers in amending or improving the present conventional agricultural paradigm with more sustainable methods, with concerns that conventional approaches are not conducive to long-term soil stability due to impacts on flora and fauna that provision the soil with vital ecosystem services. Our research has implicated some of the impacts of conventional agriculture within an Indiana context in terms of the Collembola, an ubiquitous soil/littoral-dwelling arthropod involved in decomposition, in which conventional and/or monocultural sites had consistently lower Collembolan abundance compared to agroecologically-managed fields. With these results, we still aim to assess the Collembolan potential as bioindicators within an agroconomical context once the data for our second sampling year is compiled, with the hope that such an index can help guide and support land management decisions at the policymaker and stakeholder level that would foster soil biodiversity and resulting aspects of soil fertility.
Further insight was gained as to how soil communities respond to different types of agricultural land management. In this case, farms that could be classified as "agroecological" for their incorporation of ostensibly "sustainable" or low-impact methods were compared against conventional systems to assess the impact on Collembolan communties and a few general measures of soil fertility. It was found that Collembolan communities were more abundant amongst agroecological landscapes, and ammonia, labile carbon,and soil moisture percentage were likewise higher in the agroecological fields, indicative of a greater and more stable availability of soil organic matter. Agroecological communities also exhibited slightly-more structure in terms of autocorrelation than those found in conventional landscapes, though specifically as regards to the polycultural sites, which may be indicative of landscapes that are less-disturbed in order to make greater use of ecosystem services (such as the small polycultural farms) have communities that are more spatially-structured/"orderly" than those found in more-disturbed landscapes (such as under monocultural cultivation), where there may be more frequent species turnover due to the instabilities associated with frequent disturbance. More research should be conducted with more farms recruited to further clarify whether the same phenomena is present in these systems, and our lab is eager to continue exploring the topic.