Final report for GNE19-211
Project Information
At the forefront of apicultural research, scientists are looking for ways to mitigate the high levels of colony loss, both over winter and annually. Though there are many ways to approach the main drivers of these losses (poor nutrition, pesticides, and parasites), few studies have investigated how tissue level changes relate to mortality in honey bees or their colonies. This project enhanced existing work designed to use pathophysiology as a tool for predicting overwinter colony mortality. This was achieved by combining the methods of previous work, where pathophysiology was used to successfully identify symptoms in bees and colonies diagnosed with Colony Collapse Disorder, with modern statistical techniques. To date, I have performed over 5,000 autopsies using archived samples from an existing honey bee health monitoring program. By combining the resulting autopsy data with measures of colony health from the monitoring program, I have developed a model that predicts overwinter success or failure with an overall precision of 89%. I have also used this data to investigate changes at the tissue level of individual honey bees preparing to overwinter, which may be indicators of changes to colony health that lead to overwinter mortality. This work has the potential to either reduce overwinter colony loss or reduce the capital invested in maintaining colonies over the winter. The major challenges to this effort were the refinement of the statistical models and the labor required to generate the pathophysiology data. Overall, changes in the behavior of some pathophysiology traits across years was unexpected, but this creates new questions, some of which might be addressed by the data generated from this work.
1. Perform a retrospective case control study on pathophysiology traits of bees collected from colonies in September which survived the winter or did not.
2. Further refine and validate a colony survival predictive model.
The purpose of this project is to help beekeepers mitigate colony loss. Overwinter colony losses have averaged 30% over the past 10 years, which is double that deemed acceptable by beekeepers. As it is difficult, if not impossible, to diagnose ill colonies prior to loss, my goal is to develop new tools to provide beekeepers with better insight regarding the health of their colonies. I achieved this goal by combining pathophysiological analysis with Computer Aided Diagnosis and developed a model that predicts overwinter colony loss in the fall. These tools may provide beekeepers with the information necessary to expect certain losses, thus reducing their labor and materials costs for overwinter care. They also lay the groundwork for researchers to investigate the putative causes of predictive pathophysiological states, which may lead to better tools in the future.
Research
Honey bees
Subsamples from the Sentinel Apiary program were utilized based on the criteria of paired colonies from the same apiaries; one which survived the winter and one that did not. For the sample years of 2017 and 2018 in the sample months of September, 50 pairs of colonies per year were selected and 20 bees from each sample were autopsied, for a total of 4,000 autopsies across 200 samples. All geographic regions and management practices represented by Sentinel Apiary participants were included. This retrospective case controlled experimental design is the one of the most powerful ways to make predictive models.
Pathophysiology
Autopsies were performed using standardized methods. In previous studies 17 physiological differences were assessed. In addition, I monitored two additional traits; quantity of fat body and hypopharyngeal gland condition. Recent and past work have implicated the importance of these organs in monitoring colony health, and so I included these, as they may help increase the precision of our predictive models. Twenty bees, randomly selected from each stored sub sample were autopsied, and required an average 2 hours to complete. Data collection was carried out as a binary response to the presence or absence of each trait. The resulting data set was combined with all existing data from the Sentinel Apiary program including parasite loads (Varroa and Nosema), location, number of frames of bees, brood pattern, queen status, survivorship, and any applied management practices.
Statistical Analysis
All analysis was carried out in R statistical programming language. The original work on Pathophysiology and Colony Collapse Disorder successfully classified colonies and apiaries using a statistical learning technique called Classification and Regression Trees (CART). This is a non-linear and non-parametric method that recursively divides the predictor space based on the response variable. Where these divisions are made is decided by the algorithm through minimizing the Gini coefficient, a calculated measure of equality, at each split. The output is often an easy to interpret decision tree, where the highest branch (or first split/node) is the variable with the smallest Gini coefficient. Given the right set of variables and enough observations, CART can be very useful for determining the relationship between many predictors and a response variable. These trees are, however, extremely sensitive to outliers, which can lead to misclassification, instability, or misinterpretation of the results.
To improve upon the predictive accuracy of CART, ensemble learning methods that combine the construction of many trees were applied. Such methods include:
• Bagging
o Bootstrap aggregation of multiple trees and taking their average
• Random Forests
o Like Bagging but only uses a subset of variables to build each tree before averaging
o Can reveal important relationships among underrepresented predictors and the response
• Boosting
o Each tree is built upon the error of its predecessor with the goal of reducing the error at each iteration
o The result is potentially a tree of low error with high prediction accuracy
I have applied all these methods to our current dataset to determine which performs with the highest accuracy. In each instance, two tiers of cross validation were used to assess each model: 10-fold cross validation on the entire data set and Leave-One-Out cross validation, where each colony was a single observation. In its current form, the data performed well with all methods listed but the lowest error occurs when using a form of Boosting called Adaptive Boosting, or AdaBoost, which combines a series of weak learners to produce a strong learner through iterative error reduction.
Upon completion of the proposed autopsies, I incorporated the new data with my existing set and revisited all the above methods, tuning and assessing each model accordingly. Selection of the final model was be based on the highest and most consistent prediction accuracy through the described methods of cross validation.
Results
Archived honey bee samples from the 2017, 2018, and 2019 seasons were selected for this work based on availability and completeness of management data. Overall, an additional 216 samples autopsied and the resulting data were added to the existing pool of 32 samples from the 2017 season, bringing the total to 248 samples. All autopsy data (19 variables) were combined with seasonal management (32 variables) and colony provenance data(3 variables). For the first objective of this work, only the autopsy data was used in the analysis, while all variable groups, alone or in combination, were used in the second objective. Any strongly correlated variables (>0.60) were removed from the variable pool.
Objective 1 of this proposal was to perform a retrospective case control study on pathophysiology traits of bees collected from colonies in September which survived the winter or did not. The relationships between individual pathophysiological traits and overwinter survivorship were examined using generalized linear mixed models, with each trait as the fixed effect, survivorship outcome as the response, and beekeeper nested in climate zone as the random effect. Traits that showed significant relationships then underwent relative risk analysis and were plotted against the same traits from other years (Figure 1). Risk ratios greater than 1 indicate an increased risk, while risk less than 1 indicates a decreased risk.
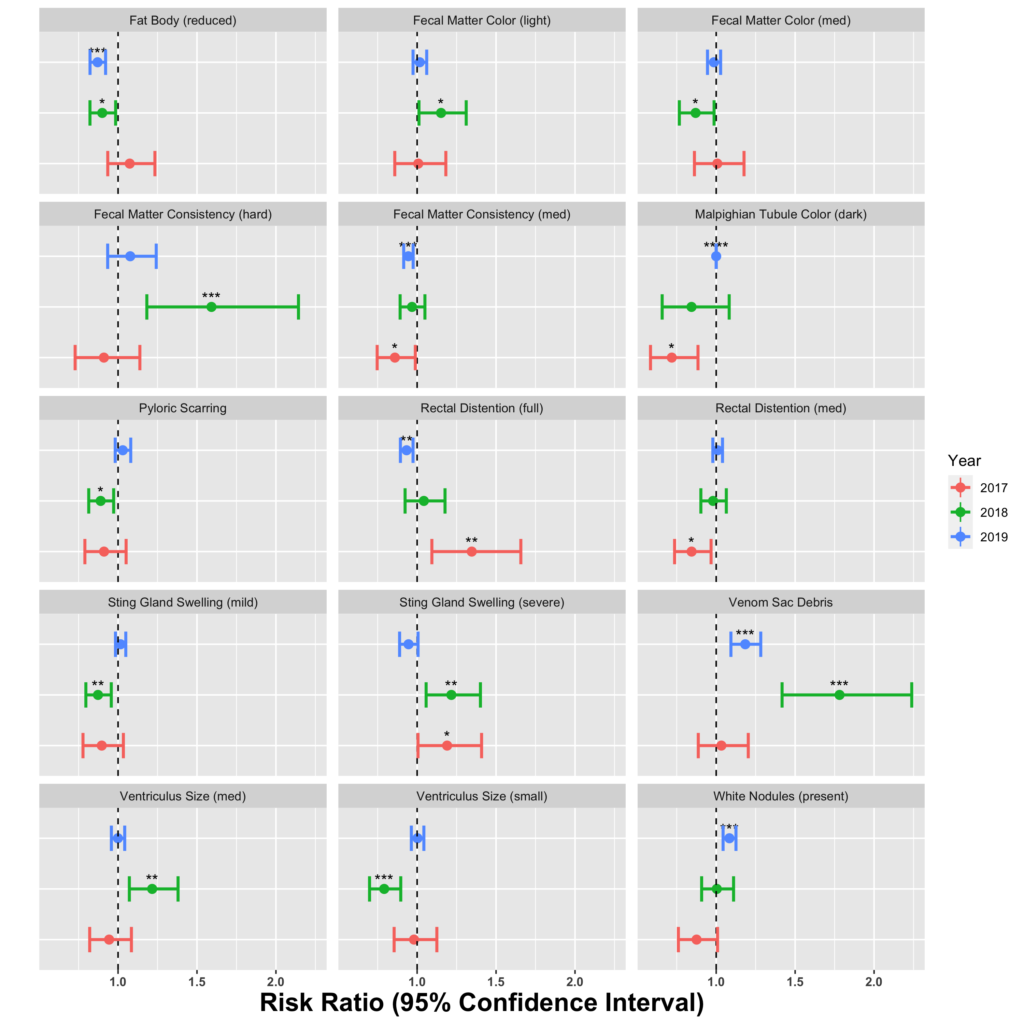
Objective 2 of this proposal was to further refine and validate a colony survival predictive model. All potential variables groups were combined and used, alone or in combination, as input for the predictive models generated. Further, data were analyzed separately per year and all potential combinations therein. Model selection was based on maximizing the sensitivity and specificity of prediction against unseen data. Those models were then further refined through cross validation and tuning of hyperparameters. Most models performed similarly to the original with an average sensitivity and specificity of 70%, with the exception of combining all years together that only achieved a sensitivity and specificity of about 63%. Further tuning and model refinement is still underway. The best model by far made use of the 2019 sample data for autopsies combined with management practices and colony health measures, achieving a sensitivity (survived the winter) of 93% and a specificity (did not survive the winter) of 75%. The model was generated using the AdaBoost Classifier and the top 10 variables important to this success are plotted in Figure 2.
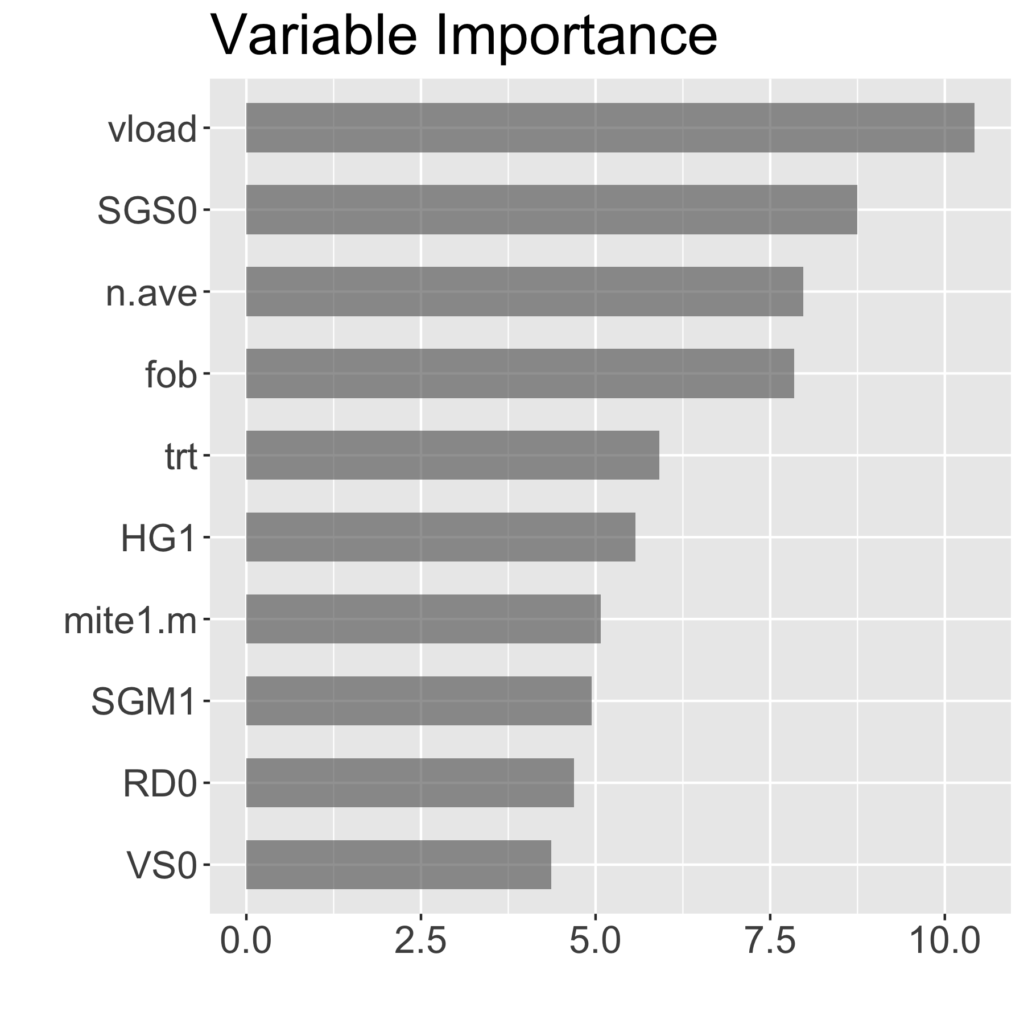
Discussion
The retrospective case control study identified several traits, which may be indicators of changes in colony health or dynamics that can lead to overwinter failure or success. For example, fecal matter of medium consistency displayed a decreased risk on average while hard consistency was seemingly the opposite. This may be in relation to the the types of solid foods being consumed or the frequency of defecation possible during periods of inclement weather. Some traits did not trend as expected, such as Sting Gland Swelling (severe) or White Nodules (present), and reversed their risk over the three year study. Interaction with other factors likely the cause of this role reversal and further investigation into the data are currently underway in an attempt to understand this possibility. It may also be that the increase in sample size over the three years produced an observable shift in these relationships.
The predictive modeling results are very encouraging and provide a path to reducing overwinter colony loss, as well as understanding relationships between new and standard methods of measuring colony health. The models agree with existing knowledge, such as Varroa being an important predictor of colony health, while adding new knowledge about changes in honey bee physiology that coincide with standard measures. This may allow future research to delve further into those relationships and how we may benefit from them. The predictive aspect of the models were either consistent or improved from the original that prompted this work. This indicates that adding observations to the data is more likely to improve the accuracy of such predictions but also that additional variables may be required to further refine and improve the models. As such, I am continuing to work toward a higher accuracy through new approaches in statistical methodology and seeking additional variables based on geographic location, such as land use and weather history.
The major achievement of this work is the continued development of a model that successfully predicts overwinter success or failure of honey bee colonies. Such a model could change the way beekeepers manage their colonies as they prepare to overwinter. As we continue to refine this model, we will be able to understand the importance of variables key to the prediction of overwinter success and one day offer recommendations based on the measure of individual colonies. Additionally, we have identified several changes in honey bee pathophysiology that may be indicators of risk for overwinter mortality. This opens new avenues of research important to understanding the dynamics of colony health.
Education & Outreach Activities and Participation Summary
Participation Summary:
To date, I have given two professional talks to bee groups in the United States and have an additional two planned for the 2020 year. Also by the end of 2020, I will be publishing a detailed blog post regarding this work on the Bee Informed Partnership website. The publication associated with this work is planned for 2021, as I am hoping to further refine the predictive modeling prior to publication.
Project Outcomes
Ideally these predictive health models will be used to either reduce colony loss through mitigated management techniques or the cost associated with managing colonies that otherwise would not survive. For commercial beekeepers, a colony that survives the winter is estimated at around $450. Extrapolated to an operation of 1000+ colonies, the economic benefit could be quite large, depending on the number of colonies at risk. Further, if it is known a colony will not survive the winter and no mitigation is possible, the cost associated with labor and feed will be retained.
The knowledge obtained demonstrated the efficacy of the methods proposed, in that predictive health modeling may be as applicable to honey bee colonies as is it to humans. Currently we have obtained a USDA grant to validate and build upon this work. We will be generating overwinter mortality predictions and testing the effects of changes to management practices based on those predictions. Other work includes tracking pathophysiological traits over time in a colony setting, in order to better understand how these traits behave under normal conditions.
Using deep learning and artificial intelligence statistical methods to generate predictive health models are relatively new in general, but currently not in use regarding honey bees. Without access to such methods and the computing power required, this project would not have been possible. Another aspect was the tremendous amount of physical labor required to collect the pathophysiology data. The current amount of time required to generate such data make deployment of such methods impractical, so additional analysis and techniques dedicated to reducing the time to collect data are necessary. As such, I am also working on methods that may automate the data collection process with minimal human interaction. Additionally, I will continue to refine the predictive models and required methodology to ensure field readiness at such a time when data collection becomes semi-automated.