Final report for GNE20-239
Project Information
Pseudomonas syringae pv. tomato (pto), the causal agent of bacterial speck of tomato, is an increasing problem in the Northeast and Mid-Atlantic region that can cause devastating crop losses. With the wide-spread emergence of chemical resistance in bacteria coupled with increasing severe weather events that favor the disease development, there is a need to find reliable long-term management strategies. One potential solution is by using bacteriocins. Bacteriocins are proteinaceous antimicrobial compounds bacteria use to kill closely related bacteria and are considered promising and safe potential agricultural bactericides and biological control agents. They could provide a sustainable alternative or complimentary strategy for managing problematic bacterial diseases in both conventional and organic systems. Current biological control strategies ignore complex community interactions, and therefore fail to produce reliable outcomes. In order to understand the role bacteriocins play in the development of bacterial speck of tomato, the bacteriocins must be accurately identified and characterized. Therefore, a bioinformatic pipeline accurately identifying the s-type bacteriocins was designed. The resulting annotation and characterization from the pipeline was used to design and execute experiments that analyze competitive interactions that correlate to increased disease development. The information gained from the pipeline and completion of the projects objectives will contribute to future study where the data can be applied to determine how and when to manipulate the foliar microbial community to effectively control the pathogen during critical points of the disease cycle for future microbial community-centered management strategies. The bioinformatic pipeline can be applied to identify and characterize all bacteriocins, not just those found in P. syringae. The pipeline will contribute to providing researchers with information needed to investigate bacteriocins as an alternative to more traditional disease management practices.
The impact of this research has yet to be seen, but the knowledge gained from the objectives of this project are far reaching and can serve as a blueprint for all bacterial plant pathogens in both disease management and antimicrobial compound analysis.
The specific objectives of this project are:
- Differentiating and classifying the S-type bacteriocins found in Pseudomonas syringaetomato (pto), by designing a bioinformatic pipeline to accurately annotate the s-type bacteriocins found in the Pseudomonas syringae species complex.
- Determine targets of individual bacteriocins in pto’sExpected outcome: This objective will identify the pathogen’s bacteriocin targets in order to understand how to limit these advantages in a community. I hypothesize that by identifying mutants resistant to knockout strains of pto that contain only 1 bacteriocin, I will be able to identify key components of the genome that contribute to mitigating competitive advantages within the context of a community.
- Assess level of foliar disease symptoms in tomato plants and determine the change in microbial community composition due to individual bacteriocins, after transplant communities are introduced to plants that have first been inoculated with pto. The purpose of this objective is to identify pto bacteriocins that contribute to disease severity of bacterial speck of tomato and identify microbial community changes due to pto bacteriocins after pto has penetrated the surface of the leaf. I hypothesize that individual bacteriocins will significantly contribute to different amounts of disease symptoms and to changes in community composition after pto has penetrated the leaf surface. (DELAYED)
- Assess the level of foliar disease symptoms in tomato plants and determine the change in community composition due to individual bacteriocins after pto is introduced to a plant that has an established microbial transplant community. The purpose of this objective is to identify pto bacteriocins that contribute to disease severity of bacterial speck of tomato and identify microbial community changes due to pto bacteriocins before pto has penetrated the leaf surface. I hypothesize that individual bacteriocins will significantly contribute to different amounts of disease symptoms and to changes in community composition before pto has penetrated the leaf surface. (DELAYED)
Added objective justification: Objective 1: Differentiating and classifying the s-type bacteriocins found in Pseudomonas syringae pv. tomato, by designing a bioinformatic pipeline to accurately annotate the s-type bacteriocins found in the Pseudomonas syringae species complex.
Upon further investigation of the BAGEL 4 results obtained from the preliminary data reported for this proposal, errors in the annotation methods were found to be present in the database that was used to identify the s-type bacteriocin content in P. syringae. The annotations provided by Bagel4 produced insufficient resolution need to carry out experiments with the P. syringae pv. tomato strains collected for this study. Before we can understand how the bacteriocins of P. syringae mediate disease and alter the microbial community, we must first accurately classify the diverse array of s-type bacteriocins that occur in P. syringae genomes. Therefore, an objective added to the proposal includes designing a bioinformatic pipeline that provides the resolution necessary to identify the s-type bacteriocins in P. syringae pv. tomato.
Current methods failed to provide accurate annotations to continue this work for the following reasons:
- Database heavily influenced by human pathogen sequences.
Human pathogen bias in the Bagel4 database is problematic for several reasons. First, human pathogens like Escherichia Coli and Pseudomonas aeruginosa that dominate the bagel 4 database for s-type like bacteriocins very rarely carry multiple s-type like bacteriocins in their genomes at one time. In fact, an Australian study done examined 266 strains of human isolated Escherichia coli found that only 38% produced any type of bacteriocin at all. Only 24 strains produced an s-type like bacteriocin, so out of 266 strains a little under 1% produced s-type like bacteriocins at all (citation). For comparison, data I collected by mining 86 publicly available P. syringae genomes for s-type like bacteriocins, I found ~37% of strains contained more than one s-type bacteriocin their genomes and 96% contained at least one s-type bacteriocin in their genomes. P. syringae pv. tomato strains encode for 2-3 s-type bacteriocins in their genomes. The volume and ubiquity of s-type bacteriocins present in P. syringae is more diverse than the range of s-type like bacteriocins found in the human pathogens that populate the Bagel4 database. Therefore, it is an inadequate reference library to use for annotation of the s-type bacteriocins found in P. syringae leading to poor resolution where significantly different s-type bacteriocins are labeled as one.
Additionally, 35% of the 97 strains used for annotating s-type like bacteriocins in the Bagel4 database originate from E. coli alone. The heavy influence of E. coli in the Bagel4 database is particularly problematic because the arrangement of the protein domains of the s-type like bacteriocins in each species is different. The receptor binding and translocation domains are switched (figure 1). The difference in domain architecture leads to poor alignments during the annotation process when the entire three domain sequence is being considered.
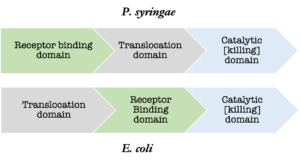
- Annotations based off partial and complete bacteriocin sequences.
The Bagel4 database is inconsistent in structure and uses both complete 3 domain s-type bacteriocin sequences and partial bacteriocin sequences that are missing entire domains. Annotations given are skewed towards partial sequences because the alignments for final annotation rely on percent identity and aren’t factoring in potential gaps or incongruence present in the missing sequence. I found specific examples where bacteriocins found in P. syrinage were annotated as a bacteriocin defined by a partial sequence but would have been annotated as a different bacteriocin if the entire database consisted of bacteriocins defined by partial sequences similar in length to the resulting annotation or complete bacteriocin sequences. Thus, the inconsistent structure of the s-type like bacteriocin sequences within the Bagel4 database results in imprecise annotations influenced by sequence length rather than overall homology.
The purpose of this project was to determine targets of bacteriocin repertoires in complex microbial communities and assess when bacteriocins are beneficial for disease development to help create future biocontrol management strategies that reduce foliar disease symptoms in tomato.
Pseudomonas syringae pv. tomato (pto), the causal agent of bacterial speck of tomato, is an increasing problem in the Northeast and Mid-Atlantic region. Managing bacterial speck in the Northeast requires a season long proactive chemical and cultural management approach (Bogash, 2016) However, current chemical control measures (e.g. fixed copper) are becoming less reliable due to wide-spread resistance in pathogen populations (Obradovic, Jones, Momol, Balogh, & Olson, 2004; Stall & Thayer, 1962). The emergence of resistance coupled with increasing severe weather events that favor disease development, have made alternative control measures, like biological control agents (BCAs), a top priority for future tomato production.
Bacteriocins are one promising biological control option for foliar bacterial diseases (Riley & Wertz, 2002). Bacteriocins are toxic proteinaceous compounds that bacteria use to kill closely related bacteria. They are attractive options for BCAs because of their narrow target range, thus, avoiding off-target effects, such as killing beneficial community members, as well as not selecting for highly transmissible resistance genes as broad-spectrum biocides do. They are also deemed safe for human consumption, because they are easily degraded by human gut enzymes (Balciunas & Martinez, 2013). Currently, the use of bacteriocins as bactericides is widespread in the food preservation industry but hasn’t been duplicated in other industries like agriculture. Current BCAs in agriculture contain one or few distinct organisms (Johnson, 2010). Inconsistent efficacy of those BCAs can be attributed to the dynamic outcome of complex competition within their microbial community. For instance, many bacterial genomes are predicted to encode multiple bacteriocins, potentially allowing for the targeting of multiple competitors and/or multiple ways to target a single competitor by the producing strain. This suggests complex interactions are contributing to the outcomes of microbial competition due to bacteriocin content. Consequently, individual bacteriocin-producing BCAs may fail to thrive long term because of other dynamic interactions within the community or fail to outcompete the pathogen during critical points of the disease cycle in which the pathogen contains other advantages (Foster, 2017).
Understanding the role of bacteriocins in mediating foliar disease symptoms within a community context can lead to microbial community manipulation by either direct bacteriocin application or by the application of BCA cocktails at key time points during the disease cycle of the pathogen Therefore, I propose to use a metagenomic approach using different microbial communities to determine the target organisms of pto’s bacteriocin repertoire and assess when during the disease cycle the bacteriocins are providing substantial advantages to the pathogen. These data will help determine which organisms are critical competitors and detect key time points for intervention. The results can be incorporated into sustainable systems of both conventional and organic tomato production to support the long-term health of tomato agricultural systems
Citations:
Balciunas, E. M., & Martinez, F. C. (2013). Novel biotechnological applications of bacteriocins: a review. Food Control(32), 134-132.
Bogash, S. (2016). Farming like You Expect Bacterial Diseases. Retrieved from PSU Extension: https://extension.psu.edu/farming-like-you-expect-bacterial-diseases
Castaneda, L. E., Miura, T., Sanchez, R., & Barbosa, O. (2018). Effects of agricultural management on phyllosphere fungal diversity in vineyards and the association with adjacent native forests. PeerJ.
Department of the Army. (2015). Intelligence Support to Urban Operations. Washington D.C.
Dorosky, R. J., Pierson, L. S., & Pierson, E. A. (2018). Pseudomonas chlororaphis Produces Multiple R-Tailocin Particles That Broaden the Killing Spectrum and Contribute to Persistence in Rhizosphere Communities. 84(18).
Foster, K. R. (2017). The evolution of the host microbiome as an ecosystem on a leash.
Granato, E. T., Foster, K. R., & Meiller-Legrand, T. A. (2019). The Evolution and Ecology of Bacterial Warfare. 29(11).
Grinter, R., Milner, J., & Walker, D. (2012). Bacteriocins active against plant pathogenic bacteria. Biochemical Society Transcript, 1498-1502.
Gugino, B. (2015). Bacterial Spot of Tomato: Biology and Management. Retrieved from PSU extension: https://extension.psu.edu/bacterial-spot-of-tomato-biology-and-management
Hirano, S. S., & Upper, C. D. (1983). Ecology and Epidemiology of Foliar Bacterial Plant Pathogens. Annual Review of Phytopathology(21), 243-270.
Hockett, K. L., & Baltrus, D. A. (2017). Use of the Soft-agar Overlay Technique to Screen for Bacterially Produced Inhibitory Compounds. Journal of Visualized Experiments(119), e55064.
Johnson, K. (2010). Pathogen Refuge: A Key to Understanding Biological Control. Annual Review of Phytopathology, 48(1), 141-160.
Mallon, C. A., & Le Roux, G. E. (2018). The Impact of Failure: Unsuccessful Bacterial Invasions Steer the Soil Microbial Community Away from the Invader’s Niche. ISME Journal, 12(3), 728-741.
Obradovic, A., Jones, J. B., Momol, M. T., Balogh, B., & Olson, S. M. (2004). Management of Tomato Bacterial Spot in the Field by Foliar Applications of Bacteriophages and SAR Inducers. Plant Disease, 7(88), 736-740.
Principe, A., Franandez, M. T., Godino, A., & Fischer, S. (2018). Effectiveness of tailocins produced by Pseudomonas fluorescens SF4c in controlling the bacterial-spot disease in tomatoes caused by Xanthomonas vesicatoria. Microbiological Research, 94-102.
Riley, M. A., & Wertz, J. E. (2002). Bacteriocins: Evolution, Ecology, and Application. Annual Review of Microbiology, 56(1), 117-137.
Stall, R. E., & Thayer, P. L. (1962). Streptomycin Resistance of the Bacterial Spot Pathogen and Control with Streptomycin. Plant Disease, 389-392.
USDA-AMS. (2017). Tomatoes. Retrieved from USDA-AMS: http://www.agmrc.org/commodities-products/vegetables/tomatoes
Williams, T. R., & Marco, M. L. (2014). Phyllosphere Microbiota Composition and Microbial Community Transplantation on Lettuce Plants Grown Indoors. 5(4).
Research
OBJECTIVE ONE:
Strains: The strains used in chapter 1 are 20 publicly available Pseudomonas syringae pv. tomato genomes, 2 Hockett lab sequenced pto strains collected by Christine Smart from tomato growing commercial settings in New York state, and 18 publicly available genomes that represent strains from 8 of the 13 phylogroups in the Pseudomonas syringae species complex. The 18 publicly available P. syrinage strains were selected from those available that were publicly available from the 2014 phylogenetic diversity study done by Berge et al. and selected to represent a diverse selection of strains in the species complex. The accuracy of the realphy whole genome multiple reference sequence alignment and tree building pipeline that will be used to describe the distribution of STBs in the species complex was performed using a data set of P. syringae strains from a 2011 comparative genomics study performed by Baltrus et al. The P.syringae whole genome tree will be constructed using the web server program T-Rex by inputting the reference alignment created by the Realphy multiple reference alignment pipeline. T-rex was used for tree building instead of Realphy, because Realphy uses a fastML method, and its output doesn’t contain the standard 1000 bootstrap support. T-Rex performs the more computationally intensive standard maximum likelihood tree output with 1000 bootstrap support.
Pipeline for STB Annotation Steps 1-7 (figure 2):
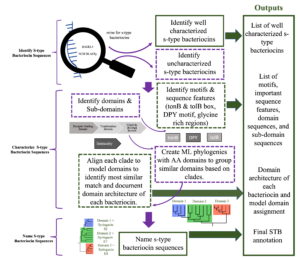
Step 1: Identifying STB sequences-
Every genome was processed using the web server application BAGEL4 to identify STB bacteriocin sequences. Amino Acid (AA) STB sequences and immunity proteins were identified via BAGEL4 and will be extracted and saved in Genious Prime and on the 2 TB hard drive. Next, each genome is blasted against a list of model and previously extracted catalytic domains and immunity proteins using the Genious Prime NCBI BLAST plug in to BLAST against each STB sequence. Settings are adjusted to retrieve sequences that are 50% or more identical for the catalytic domain, and 30% or more identical with the immunity protein to confirm BAGEL4 results and to identify STBs not detected by BAGEL4 (a common problem that occurs with some genomes that were previously annotated before deposited in NCBI). Each STB match is then extracted and stored into Genious prime and on the 2TB hard drive. Due to the prior research done by Martin Ghequire’s lab (Ghequire & De Mot, 2014) (Ghequire & Öztürk, 2018) (Ghequire, Swings, Michiels, Buchanan, & De Mot, 2018), STBs that are identified as PaeM (in BAGEL4) and/or extracted with the Genious Prime NCBI BLAST plug in that are 70% identical with the catalytic domain sequences listed as M will be identified as syringacin M and will be considered well characterized. All the other sequences that are extracted will continue to be processed for STB characterization and naming.
Step 2: Identify receptor binding, translocation, catalytic/killing, and immunity protein domains-
Every sequence will be annotated using the Genious plug in for Interpro scan to identify any conserved protein domains, disordered regions, and coiled coils. Receptor binding domain sequences will be identified starting from the first amino acid through the coiled coil region (the coiled coil region is a well-documented characteristic for receptor and/or translocator binding both at the beginning and at the end of a receptor binding domain) or it will be identified by the start of an identified conserved domain translocation region if interproscan was unable to identify a coiled coil region 100AA+ into the sequence. Translocation domain is identified by the identification of a conserved translocation domain region via interproscan or will start where the receptor binding domain ends and end at the start of the catalytic domain. Catalytic domain is identified by conserved protein domains found by interproscan or will start at the end of an identified translocation domain and the end of the bacteriocin sequence. Immunity proteins are identified by BAGEL 4 and NCBI BLAST when identifying STB sequences in the previous step.
Step 3: Identify putative DPY motifs, tolB boxes, and TonB boxes-
DPY motif: The DPY motif was identified by using the Genious Prime “find motif” function with the input D[X5]P[X8-X9]Y against each uncharacterized sequence. DPY motifs found in ecoli are D[X5]P[X8]Y and were described by Sharp et al., 2017. However, after reviewing STBs of in P. syringae STBs, I found they occasionally have an extra AA between P and Y and adjusted the motif to reflect that observation. The motifs were described by Sharp et al (2017).
TolB box: TolB boxes were identified using the Genious prime “find motif” function with the inputs DGTXWSSE and G[X4]GW[X6]W based on motifs described in Zhang et. Al, 2009.
TonB box: Multiple sequence alignments were made using Blosum62 global alignment of the first 60 amino acid bacteriocin sequences in geneious and the model TonB sequences. Putative TonB boxes identified will be identified as the 10AA sequence that is identified by overlapping sections of the alignment.
Step 4: Make ML phylogenies with each catalytic, translocation, and immunity protein domain and identify clades that share atleast 70% homology-
Multiple sequence alignments will be made using Blosum62 global alignment of amino acid sequences in geneious. Phlogenetic trees will be constructed using PhyMLwith a Le Gascuel substitution model, and branch support calculated with 1000 bootstrap replicates, optimizing for topology, length, and rate. Clades where all members are 70% or more identical will be considered as one individual domain and given a temporary clade identification number (CIN) starting with a letter that corresponds to domain type for naming and architecture identification. (e.g. C4 = catalytic domain clade 4, T2 = Translocation Domain clade 2). Preliminary data for this step can be viewed in Preliminary figure 2 and 3 on pages 20 and 21.
Step 5: Identify domain architecture, arrangement of domains, of each sequence-
Domain architecture or the arrangement of the domains for each bacteriocin is identified by recording the CINs for each of the receptor binding, translocation, and catalytic domains. (e.g. R2, T1, C4).
Step 6: Rename catalytic CIN domains based on closest homology to model catalytic domains-
Each catalytic CIN will be given a new name that corresponds with it’s homology to model catalytic domains except syringacin M. Homology will be determined by performing Blosum62 pairwise local alignments using the Smith-Waterman algorithm of member of a CIN with every model catalytic domain listed in table 3 except syringacin M. The catalytic domain that shares the most homology with all the members will become the Model Catalytic Domain Homology (MCDH) and replace the CIN. (e.g. C1=E9, C2=S2, C3=E8).
Step 7: Final name assignment-
Last, final names are assigned first based off of the MCDH. Then finally determined by the architecture of the domains. For example, if two E8 MCDH contain different translocation domain CINs, then one will be Named Syringacin E8a and the other Syringacin E8b.
OBJECTIVE TWO:
Knockout Strains: Pto strain, PT23, will be used as the model for this experiment. First, the R-type bacteriocin will be knocked out of KLH279 (KLH279-R) and KLH279-R is what will be used as the background for the rest of the clones. This is to ensure that the R-type bacteriocin isn’t influencing the observations throughout the experiment. Knockouts (KO) will be made by an overlap extension using the method. Deleting the bacteriocins identified in bacteriocin strain KLH279 obtained from the bioinformatic pipeline designed in objective one and from preliminary data previously collected from mining 25 publicly available pto strains and the sequences of 56 pto strains sequenced by the Hocket lab for bacteriocins. PT23 carries one R-type tailocin, and two STBs (syringacin H1 & syringacin D). The STBs will be referred to as bacteriocins A and B.. Knockouts will be made from KLH279-R, that only contain one STB each. Then one knockout will be made that doesn’t contain any bacteriocins. Strains used for this study will be as follows KLH279-R, KLH279+A, and KLH279. With KLH279-R as the positive control, KLH279-AB and a distilled water with buffer as negative controls.
First, the sequence flanking the targeted genes was amplified with Phusion High-Fidelity DNA Polymerase (ThermoFisher Scientific, Waltham, MA), separated in 1% agarose gel and purified with QIAquick Gel Extraction kit (Qiagen, Hilden, Germany). Next, the matching pair of individual flanking fragments will be cycled without primers to hybridize the internal primer tails, followed with amplification of the entire deletion gene product with BP tailing primers. All primers were designed using Geneious Prime software. It is important for the BP tail primers to be present as they are required for cloning downstream. The complete deletion gene products are excised from 1% agarose gel and purified. These fragments are recombined into the entry vector pDONR207 using BP clonase (Invitrogen, Carlsbad, CA). Gentamicin-resistant clones with the correct fragment are combined into the destination vector pMTN1907 using LR clonase (Invitrogen, Carlsbad, CA).
Following this, kanamycin-resistant clones with the correct fragment undergo plasmid DNA purification using the QIAprep Spin Miniprep Kit (Qiagen, Hilden, Germany). The mutant plasmid is then electroporated into E. coli S17-1 cells. Kanamycin resistant colonies are screened by PCR and confirmed colonies are used as the mating donor in a biparental conjugation with the desired construct of PT23. Biparental mating mixtures were plated on KB supplemented with tetracycline and nitrofurantoin. Colonies are screened by PCR and confirmed colonies are incubated overnight in KB broth amended with tetracycline. The overnight cultures are serial-diluted and plated onto KB amended with 5% (wt/vol) sucrose. Mutants retaining the desired deletion are confirmed by tetracycline sensitivity and PCR verified using gene specific primers to harbor a fragment of reduced size compared to the wild-type strain of KLH279.
Pairing knockouts with sensitive target strains: Supernatants from knockouts, KLH297+A & KLH279+B, containing one bacteriocin will be collected and soft agar inhibition test will be performed against the 56 strains of pto to pair the triple KO strains with their respective sensitive counterpart. Due to delays because of the pandemic and the time consumed pursuing the added objective this work is being conducted under a grant obtained from an ARFI-USDA NIFA Predoctoral fellowship and is still ongoing.
Mutant Selection: Once the bacteriocin producing strain has been paired with the bacteriocin sensitive strain, then selection of bacteriocin mutants by mixing 50uL of the susceptible strain in log phase grown in KB 50uL of supernatant supernatant extracted from the 4 KO strains in a microcentrifuge tube (using a sterile KB as a control). After, vortexing the tube for 10 seconds I will let the supernatant incubate for 30 minutes at room temperature vortexing for an additional 10 seconds every 10 minutes. Following incubation, a 1/10 serial dilution series by spotting the mixture onto non-selective KB plate. Then the dilution will incubate on the plate at 26 degrees C for 24-48 hours. Finally, individual resistant colonies should emerge at one of the dilution points and will be picked and whole genome sequenced using Illumina to identify genetic mutations that indicate resistance by comparing the mutants to the wild type whole genome sequences that were assembled in April of 2020Due to delays because of the pandemic and the time consumed pursuing the added objective this work is being conducted under a grant obtained from an ARFI-USDA NIFA Predoctoral fellowship and is still ongoing.
Microbial Community Collections: Two plant communities plant selected microbial communities 1 month prior to the start of objective 3, collected and kept at 4°C for the experiments to be performed for this project in 2021 and 2022. Leaf microbial community samples will be taken from tomato plants grown at the Pennsylvania State University research farm that are not undergoing experimental treatment in another study will be harvested for their and from communities on leaves from a non-agricultural native plant at least 500 feet away from managed land. Then they will be stored for no longer that 1 month at 4°C, before they will be dried in a drying oven at 25°C and fragmented by grinding the plant material finely with a mortar and pestle. Due to delays because of the pandemic and the time consumed pursuing the added objective this work is being conducted under a grant obtained from an ARFI-USDA NIFA Predoctoral fellowship and is still ongoing.
OBJECTIVE THREE: Due to delays because of the pandemic and the time consumed pursuing the added objective this work is being conducted under a grant obtained from an ARFI-USDA NIFA Predoctoral fellowship and is still ongoing.
- Design: A randomized block design will be conducted blocking by the source of the starting microbial community (tomato vs. non-tomato). All objective 2 treatment groups will be considered “invading”. Invading treatment groups will have the bacteriocin producing strains applied as a foliar application after transplant communities have 72 hours to establish. Invading treatment groups are measuring the benefits that bacteriocins provide during colonization of an established community. This is important for disease spread within a field. Each treatment block will consist of 6 treatment groups with 5 plants each for a total of 60 plants for objective 2.
- Metagenomic community identification: Next, I will compare the change in community compositions in all my treatments, 7 days after initial symptoms appear on the wt treatment groups, to the negative controls. Then, I will use a metagenomics approach for community profiling instead of the traditional 16SrDNA, since metagenomics provides a greater resolution of the community. This will likely be important based on knowledge of bacteriocin narrow spectrum targeting. Metagenomic comparisons will be addressed using the methods listed under Metagenomics.
- Disease assessment: Additionally, the plant will be evaluated for disease severity using the methods listed under quantitative and qualitative disease assessments and evaluated for statistical significance using the methods under statistical analysis. If there is a statistical significance to a treatment group, the experiment will be performed again with a complimented version of the significantly different strain and with the wt and KLH279-AB strains as controls in order to provide evidence that the significant differences in the community are in fact due to a specific bacteriocin in the community and are not the result of some other interaction happening on the leaf surface.
Objective 4: Due to delays because of the pandemic and the time consumed pursuing the added objective this work is being conducted under a grant obtained from an ARFI-USDA NIFA Predoctoral fellowship and is still ongoing.
- Design: A randomized block design will be conducted blocking by the source of the starting microbial community (tomato vs. non-tomato). All objective 3 treatment groups will be considered “defending”. Defending treatment groups will have the bacteriocin producing strains inoculated directly into the leaf. Then foliar application of transplant communities will be applied 72 hours after syringe inoculations. The purpose of the defending treatment groups is to measure the benefits a bacteriocin provides during establishment and persistence of the community. Each block will will consist of 6 treatment groups that contain 5 plants each for a total of 60 plants for objective 3.
- Metagenomic community identification: Next, I will compare the change in community composition 7 days after initial symptoms appear on the wt treatment groups to the negative controls using a metagenomic approach for the same reasons stated in objective 2.
- Disease assessment: Additionally, the plant will be evaluated for disease severity using the methods listed under quantitative and qualitative disease assessments and evaluated for statistical significance using the methods under statistical analysis. Finally, if there is a statistical significance within a block or between treatment groups, the experiment will be performed again with using complimented versions of the significant strains, combined with the wt and KO-ABCD strains as controls respective positive and negative controls. The purpose of this step is to provide evidence that the significant differences in the community are in fact due to a specific bacteriocin in the community.
Pathogen inoculations:
For plant inoculations, Pseudomonas syringae pv. tomato (pto) will be grown in liquid King’s B (KB) media at 28C for 24 hours. The cells are centrifuged at maximum speed, washed with 10 mM MgCl2 three times, then resuspended in 10 mM MgCl2. Bacterial suspensions are standardized to an optical density at 600 nm of 0.1 . Final inoculum will be quantified by plating serial dilutions of leaf wash onto selected media with antibiotics. At 3 weeks old, five tomato plants for all treatments will be sprayed with pathogen suspension until dripping. The plants will be lightly shaken to remove large droplets then covered with a plastic bag for 24 hours to increase relative humidity to allow for pathogen establishment. The negative control group will be the treatment group that undergoes inoculation with pto that contains a quadrupole knockout (KLH279-AB), containing no active bacteriocins, and the positive control will be inoculation with a strain from our wild type (wt) representative from collection of Smart lab isolates that won’t have any of the bacteriocins knocked out. Then all treatments will be assessed for disease severity and pathogen load will be calculated using the methods outlined below.
Metagenomic analysis:
Shotgun metagenomics will be performed on each of the treatment groups after the 72 hours after the initial transplant community or pathogen inoculation are established, then again 7 days after the first disease symptoms appear on the wild type treatment group. Reads will be assembled into contigs, then quality control will be performed to detect if sequences are too long or ambiguous. Next the contigs will be aligned to an established database and then classified by their estimated phylogenetic relationships. Last, OTUs will be defined and clustered using a 97% similarity index. Last diversity of the samples will be analyzed with Principle Coordinate Analysis using UniFrac as the similarity index in order to incorporate phylogenetic information and presence absence information into the coordinate analysis since relatedness of the bacterium plays a key role in targeting range of the bacteriocin.
Qualitative Disease Assessment:
Following inoculation of tomato plants with pathogen, all plants will be evaluated on a symptom index scale from 1 to 3 one week after symptoms develop on the plants that have been inoculated or sprayed with the pto strain without any knockouts (positive control). The disease severity scale that will be used is as followed: 1 = small spots and/or water-soaking, 0-10% leaf coverage, 2 = 20-30% leaf coverage, 3 = >30% leaf coverage. Disease severity and incidence will be calculated in comparison to the control groups. It is assumed that if disease doesn’t develop within a week of the control plants, it is unlikely to develop.
Quantitative Disease Assessment:
After the qualitative assessment of disease, pto leaf exterior populations will be dislodged from the surface of three tomato leaves per plant by submerging the leaves in tubes with 10 mM MgCl2 buffer and sonicating for 10 minutes. The leaves will then be removed from the buffer and the bacterial cells will be centrifuged and resuspended in 10 mM MgCl2 buffer. Following removal from buffer, the leaves will be surface sterilized by placing individual leaves in a beaker containing 150 mL of 10% (vol/vol) bleach solution for 5 minutes with gentle shaking. For the enumeration of the leaf interior pto population, the leaves will be rinsed in sterile distilled water and dried inside a laminar flow hood for 1 hour.
The leaf interior populations will be determined by homogenization of individual leaves in 10 mM MgCl2 by mechanical disruption. Both the exterior and interior leaf pto populations will be calculated by serial diluting onto KB media amended with selective antibiotics. The plates will then be incubated at 28°C for 24 to 48 hours. The colonies that formed after incubation will be counted and transformed into the number of colony-forming units per leaf. This approach will add a quantitative measurement to support the qualitative disease assessment.
Statistical analysis of disease:
-
Qualitative disease analysis: Objectives 2 and 3 will each be a randomized block design that is blocked by source of transplant community communities (tomato and non-tomato). Each block will undergo 6 treatments each. A positive and negative control along with 4 KOs containing only 1 bacteriocin each. Analysis of variance (ANOVA) and Tukey pairwise comparisons will be used to infer statistically significant relationships overall between the treatment groups and between the blocks. Blocking by community helps determine if the starting community contributes significantly to the effectiveness of an individual bacteriocin. Last, final data from objectives 3 & 4 will be compared using ANOVA and Tukey pairwise comparisons to compare disease severity between invading vs. defending treatments.
-
Quantitative disease analysis: For pathogen enumeration, the pathogen populations will be analyzed for normality and heteroscedasticity, and log transformed as needed. The means of the pathogen populations for both the exterior and interior part of the leaf will also be compared using ANOVA multiple comparison with a Tukey post-hoc test. All analyses will be performed by using open source software in R.
Objective 1: Creation of a bioinformatic pipeline that can accurately identify s-type bacteriocins in the pseudomonas syringae species complex.
The Pipeline:
The pipeline starts by mining P. syringae genomes for s-type bacteriocin sequences by blasting against a library of model s-type bacteriocin protein domains. The sequences are then extracted and separated into “well characterized” and “under-characterized” groups. Well characterized s-type bacteriocins are labeled Syringacin M. Syringacin M has been thoroughly defined and investigated by Martin Ghequire’s Lab. All other sequences are considered under-characterized and are then broken down into their respective domains, sub-domains and immunity proteins using protein structure prediction. Each domain is then analyzed for motifs and sequence features that are important for characterization and a list of these is documented. Additionally, each domain is analyzed for similarity and homology with other known and unknown bacteriocin domains and labeled accordingly. Next, the domains are brought back together, and the architecture of the domains is recorded as part of the output. Last, a name is assigned based on predicted killing action and domain architecture.
Discussion:
A bioinformatic pipeline was built to identify the s-type bacteriocin repertoire in P. syringae. The pipeline provides better resolution than current s-type bacteriocin annotation methods by breaking down the protein into its three domains and its immunity protein before analyzing them as separate entities before bringing the parts back together for final annotation. This solves the problem that Bagel4 has by being heavily influenced by bacteriocins that originate from E. coli, because the arrangement of the domains is not considered during the alignment process, only during the final arrangement. Additionally, analyzing the domains separately keeps the database of sequences consistent in length and structure providing alignments that aren’t biased by being aligned to sequences of random length. Domain boundaries in the pipeline are defined by predicted structural components that are important for functions of the domain. This assures that domain identification is based on function and structure rather than a program that annotates conserved domains that contain the same biases that the Bagel4 bacteriocin annotations have.
The pipeline searches for motifs and sequence features that are important for s-type bacteriocin classification and function. The output provides a list of these features. These details provide insight for questions and experimental designs associated with the bacteriocins found in silico. Ton and Tol box motifs and glycine rich extreme n-terminal regions provide information that hints to how the bacteriocin enters the target cell. Furthermore, the DPY motif, enzymatic killing action motif, and pore forming motifs predict how the bacteriocin kills its target cell. This information is not only important for classifying bacteriocins, but also for thinking about their function within a microbial community. Overall, having this list of motifs and sequence features benefits researchers that want to classify the s-type bacteriocin and hypothesize dynamic competitive interactions like those that lead to plant disease. This is the first step to understanding how s-type bacteriocins contribute to changes in microbial community composition and plant disease.
Objectives 2-4:
Due to the pandemic and the necessary addition of an objective there are no results to report on this section. However, this work is being completed with funds secured in part because of the work completed in objective one.
The key conclusion for this project is that by breaking the s-type bacteriocin sequence into its three domains and immunity protein, we are able to provide more accurate annotations that are needed to continue with objectives 2-4 of this project. Accurately characterizing s-type bacteriocins that originate environmental bacteria and plant pathogens is the first step to designing experiments that unravel how microbial interactions involving bacteriocins contribute to plant disease and how these interactions can be used to mitigate disease for farmers and/or improve the efficacy of current biocontrol applications. This pipeline has built a foundation for objectives 2-4 of this project that ask specific questions about the agriculturally significant plant disease, bacterial speck of tomato that is currently being performed. The rationale behind the design of the pipeline can be applied to s-type like bacteriocins of any species of bacteria. Therefore, the pipeline can benefit researchers interested in s-type like bacteriocin-mediated interactions of any bacterial plant pathogen by providing accurate annotations and putative motifs that help predict function.
Education & Outreach Activities and Participation Summary
Participation Summary:
Oral Presentations:
"Bioinformatic Pipeline for Annotating and Characterizing the S-type Bacteriocins present in the Pseudomonas syringae species complex" at the Penn State University, Department of Plant Pathology and Environmental Microbiology's Spring 2022 Seminar Series
Poster presentations:
"Bioinformatic pipeline for annotating the s-type bacteriocins in the Pseudomonas syringae species complex" at the Annual American Society for Microbiology ASM Microbe meeting in Washington DC 2022
Upcoming-
"Annotating the s-type bacteriocin arsenal present in the Pseudomonas syringae species complex"at the Annual American Phytopathological Society Plant Health meeting 2022
Video Presentaitons:
"Uncovering the s-type bacteriocin repertoire of P. syringae pv. tomato" at The Penn State University's 36th annual Graduate Student Exhibition 2021
"Annotating the genomicially concealed weapons of Pseudomonas syringae" at Penn State University's 37th Annual Graduate Student Exhibition 2022 *First place award*
Project Outcomes
This project is just the beginning of investigating how s-type bacteriocins contribute to disease outcomes in the phyllosphere. It is the first step to understanding how s-type bacteriocins can be leveraged for sustainable microbial community-based management practices on important vegetable crops like tomato. The bioinformatic pipeline that was designed to identify the s-type bacteriocins in Pseudomonas syringae pv. tomato can be used to identify s-type bacteriocins in all bacteria. While my specific pipeline is tailored towards bacteriocins found in the genomes of Pseudomonas syringae, the steps and pipeline design can be applied to any bacteria. It will provide more detailed outputs needed to answer questions relevant to microbial interactions. It particularly benefits research that focuses on plant and environmentally associated bacteria, by providing results that aren’t reliant upon E. coli protein domain architecture, because s-type and s-type like bacteriocins of different species vary in domain architecture.
The desired benefit for growers is to have efficacious sustainable long-term disease management options with minimal input to the field. Identifying the natural weapon repertoires of plant pathogens and investigating the roles they play in agricultural microbiomes, is filling in a gap of knowledge that is necessary for understanding why current microbial based management strategies like biocontrol agents are not efficacious. Research into interactions occurring on tomato leaves will be conducted over the next two years providing researchers and farmers with details of the interactions that occur on tomato leaves and how they contribute to disease and how they change the composition of the microbial community as a whole. More research is needed to assess the economic, environmental, and social benefits for growers.
Overall, this project has allowed a deeper understanding of the s-type bacteriocin repertoire that exists within the Pseudomonas syringae species complex. Characterizing the types of weapons that environmental bacteria and plant pathogens use to compete in their respective microbial environments is the first step to understanding how to experimentally discover how microbial interactions involving bacteriocins contribute to plant disease and how these interactions can be used to mitigate disease. This project has created a pipeline that can be used to identify s-type bacteriocins in the genomes of bacteria that lead to a wide range of plant diseases caused by Pseudomonas syringae. This information can be used to investigate microbial interactions beyond the objectives of this proposal. The future of this proposal is to investigate the objective 3 and objective 4 that is already outlined in the proposal. They will be completed in 2022-2024 from grant funding from USDA-NIFA.