Progress report for LNC22-474
Project Information
Problem:
The Driftless Area covers 24,000 square miles at the junction of four North Central States: Minnesota, Wisconsin, Iowa, and Illinois. The name indicates that the region, in comparison to the surrounding areas, lacks glacial deposits. Even though the region's glacial history is more complex, the name captures the region's strong physiographic identity. Its complex topography does not allow mechanized industrial farming, which is overwhelmingly present in the North Central states.
Farmers contributing to this proposal were eager to list topography-affected soil problems: (i) extremely diverse soil texture, moisture, and organic matter contents that limit timely field preparation, adopting ideal rotation, and fertilizer application, (ii) flooding and saturation of low-lying fields, (iii) distinct micro-climate between the ridge tops and valleys, and (iv) rapid soil erosion across the range of slopes (see collaboration letters). As the farmers’ responses highlight, it is within the individual farms that the highly variable soil conditions occur in this unique region. However, most research on soils and soil erosion focus on watershed scales. Consequently, we lack a practical guideline for small-scale farmers to optimize the sustainability and productivity of their lands according to their complex soils and topography. This issue is urgent as the Driftless Area is often seen as a magnet for small-scale farmers.
Approach:
This research project will:
- Collaborate with at least six organic vegetable farms in the Driftless Area, as organic vegetable production is one of the most common agricultural land-uses in the region. Via focus group and individual farmer interviews, we will learn how soil-topographical relationships are factored into the land-use decisions the farmers make.
- Characterize how topography affects land uses, vegetable productivity, and soil health within individual farms owned by our collaborator farmers. We will collect soil properties data and use remote sensing and high-resolution elevation data. Remote sensing-based characterization of vegetable health and yield will be made as a function of topography.
- Integrate farmer narratives and biophysical data in order to (1) document novel economic and environmental effectiveness of within-farm farmer soil-landscape use and (2) co-develop alternative models for agricultural use of complex soil-landscapes in part by applying precision agriculture principles and technologies in organic agriculture.
Relevance: All soil parameters and the agricultural impacts on soil erosion and hydrology strongly reflect topography that varies within the scales of small farms in the Driftless Area. Assessing and improving these connections are important for organic farm productivity and sustainability.
Our objectives are to: (1) better understand within-farm agricultural use of soil landscapes, and (2) to support positive land-use decisions, or propose improved or alternative within-farm agricultural use of soil landscapes and management practices by combining precision agriculture and organic agriculture.
Our expected outcomes are (1) farmers have increased knowledge and awareness about the role of topography on the productivity and sustainability in their farms and (b) farmers have plans to change or do something differently based on our research disseminated through our outreach.
In the first year, our research team focused on (1) understanding and documenting topography-related issues and (2) characterizing the topography and soils of participating organic vegetable farms.
In this report, I have included the hypotheses specific to the Year 1 activities. These hypotheses were tested through a mix of activities that included farmer focus groups, farm visits, GIS analysis, and soil sampling. These activities are described in the Research and Project Activities sections of this report.
Cooperators
Research
Year 2
In the second year, our research team focused on (1) measuring key soil properties (pH, C, and N), (2) developing methods for using remote sensing and precision ag tools to characterize within-fields and within-farms variability in soils and crop productivity, (3) completing the installation of soil temperature sensors, and (4) completing soil sampling for erosion assessments.
We hypothesized that organic farms in the Driftless Area exhibit significant variability in crop growth and significant soil variabilities.
The rugged landscapes of the Driftless Area present farmers with multiple challenges in managing the high variability of soils associated with complex topography. We focused on organic vegetable farms in this region because it has increasingly attracted organic farmers over the past decades.
Year 1
In the first year, our research team focused on (1) understanding and documenting topography-related issues and (2) characterizing the topography and soils of participating organic vegetable farms. Below are the active hypotheses we tested to address these research objectives in Year 1.
- Within the cropland of organic vegetable farms in the Driftless Area, the spatial distribution of cropland is largely determined by topography.
- There are two types of organic vegetable farms in terms of topography. The first type of farm selects and cultivates only relatively flat areas of ridge or valley bottoms. The second type of farm - without access to relatively flat areas - cultivates hillsides.
- In the first type of farm, ridge soils are more spatially and temporally variable.
- In between the two types, the farms that farm the hillsides face greater challenges of soil variability.
Year 2
For the second year, we present the materials and methods in two sections: crop growth variabilities and soil variabilities
Crop growth variabilities:
We used high-resolution satellite remote sensing to assess crop growth variability within fields and farms. We need a fast and reliable approach to evaluating field variability and implementing zone-specific management strategies to reduce inconsistencies and improve productivity. Small field sizes, diverse crops, and complex terrain in organic farms made it difficult to extract accurate and meaningful information from remote sensing data, which explains the lack of precision agriculture applications for organic vegetable farms and small farms. We found significant challenges in developing methods for assessing the spatial variability of crop growth for delineating management zones. The biggest challenge is the lack of crop growth models for vegetables grown in our collaborator's organic vegetable farms. Additionally, no yield data is available from our collaborator farms. We note this because it appears typical that most, if not all, organic vegetable farms lack yield data.
Farm B has kept a record of plot-specific vegetable varieties, planting dates, and harvest dates, which was shared with our research team. The management data further included field boundaries, tillage method, and other relevant details recorded by the farmer. Farm B managed 31 valley fields (A total of 107 ac) and 18 ridge fields (a total of 73 ac) in 2023. We combined this management data with several geospatial data. We calculated relative elevation, slope, aspect, profile curvature, plan curvature, tangential curvature, topographic wetness index, terrain ruggedness index, topographic position index, and roughness from the LIDAR data. Soil properties data were obtained from the gNATSGO database and POLARIS digital soil mapping database.
On those data sets, we overlaid crop data. We obtained high-resolution (3 m), cloud-free PlanetScope images with a daily revisit cycle during the crop growth periods provided by the Farm B. NDVI was calculated, and for each field, the NDVI during the crop vegetative growth stage was identified and standardized by computing SVI = NDVI / avgNDVI. This index reflects crop growth conditions (<1 indicates poor growth, ~1 indicates moderate growth, and >1 indicates good growth).
Soil variabilities:
Among soil properties, soil organic matter is considered one of the most important indicators of soil health and sustainability, while soil nitrogen content is critical for fertility. It has long been known that these two key soil properties vary substantially with topographic position. Therefore, we aimed to provide farmers with insights into the within-farm and within-field variability of soil carbon and nitrogen.
In the Driftless Area, in addition to topography, parent materials are a significant source of soil variability. While soils on hilltops and plateaus have developed from eolian silts, soils in the valleys and floodplains originate from fluvial deposits, and hillslope soils have formed from alkaline sedimentary rocks or colluvium. This variation in parent material and topography can significantly influence soil chemistry. Therefore, we also examined the within-farm and within-field variability of soil pH.
For the larger organic vegetable farms A and B, we examined and compared the fields on the hilltops vs. the fields on the valley floor. For the smaller farms C and D, we examined and compared the fields between erosional and depositional areas. At three farms (farms A, C, and D), we designated plots of 630m2 per landform and collected 21 composite samples to the soil depth of 20cm. The soil sampling efforts took place between the Fall of 2023 and the Summer of 2024. At one farm (Farm B), we gridded the hilltop field and valley field and conducted soil sampling. We collected a composite sample (5 sub-samples per hectare). Soil carbon and nitrogen concentrations were measured using the combustion method. The pH was also measured with CaCl₂ solution (0.02M)
Year 1
In the first year, we worked to test the hypotheses using four approaches. The first was focus group meetings with farmers, the second was farm visits, the third was geospatial analysis, and the fourth was soil sampling and laboratory analysis.
- Focus group meeting: We met with the owners and managers of the four participating organic vegetable farms in the Driftless Area. During this meeting, the farmers described the topography-related issues and challenges specific to their farms. The list generated from the meeting served as our checklist during the farm visits. I summarized the information from the meeting in the project activities.
- Farm visits: We visited the four participating organic vegetable farms. The visits were informative and confirmed many of the issues that the farmers shared with us during the focus group meeting. The visits revealed the different ways in which the farmers use or cope with the topography. We also learned that there are drastic differences between the large organic vegetable farms and the small ones in the way their fields are tied to different topographic zones. I have summarized the field visits in the project activities.
- GIS Analyses
- Google Earth: We obtained the fields and farm boundaries in Google Earth format from four farmers who participated in the focus group (2/1/2023).
- Topographic analyses: From the LIDAR data, we constructed a 3-meter DEM for the four farms. From the DEM, topographic variables such as slope, aspect, topographic position index, and topographic ruggedness index were calculated. We calculated their mean values and deviations per individual field by superimposing the field and farm boundaries on the topographic data. During the first year, method development was focused on the Featherstone farm in Minnesota.
- Soil Survey: Web soil survey data were extracted for the four farms that participated in the focus group.
- Based on the Planetscope 3-meter satellite imagery and farm visits, we began creating hypothetical management zone boundaries within which topography and soil conditions are homogeneous.
- We collected crop data from PlanetSCOPE (3m resolution, 8 bands with near infrared (885-845nm), red edge (697-713nm), red (650-680nm), green (547-583nm), green I (513-549nm), yellow (600-620nm), blue (465-515nm), coastal blue (431-452nm) for the two periods: 2023 May to September and 2022 May to NOV for all farms.
- We collected bare ground satellite images from Planetscope for 2023 Apr, March, 2022 Apr, March, Nov or Dec and 2021 Apr, March, Nov or Dec and 2020 Apr, March, Nov or Dec.
- We also collected and began organizing soil survey data per field and per farm.
- Soil Sampling:
- Based on the preliminary GIS analysis, we conducted intensive soil sampling in two topographically contrasting areas on the farm B.
- These samples will be analyzed for organic carbon and pH
Results in Year 2
Crop Variabilities
We found that the ridge fields had greater variabilities in crop growth and soil properties than the fields located in valley fields.
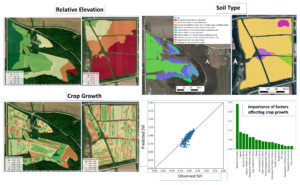
Integrating elevation, slope, and soil properties with high-resolution remote sensing data enables precise identification of spatial variability. We found that the key factors influencing crop growth include (in the order significances) relative elevation, slope, aspect, soil pH, organic matter, and topographic wetness index. We used those variables to delineate management zones for the farm. Management zones better identify areas of low and high spatial variation for crop growth compared to the landscape.
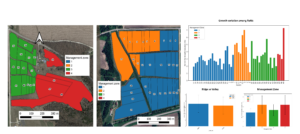
management zones (relative elevation, slope aspect, soil pH, organic matter, and topographic wetness index were used for management zone delineation)
Soil Variabilities
In the larger farms, A and B, fields are located either on relatively flat ridges or in flat valleys. In contrast, the two smaller farms, C and D, have fields extending from the backslope to the toeslope. At the larger farm A, soil pH was more acidic in the ridge fields (6.9 ± 0.1) than in the valley fields (7.2 ± 0.1). However, the opposite trend was observed in farm B (Figure 7). In the smaller farms (e.g., Farm C), soil pH also varied significantly depending on slope position, with the direction of the difference differing between farms.
The highest soil carbon content was found in the valley or lower slope fields of the larger farms (2.1 ± 0.04% at Farm B and 2.1 ± 0.02% at Farm A), followed by the ridge fields of the larger farms (1.64 ± 0.05% at Farm A and 1.56 ± 0.04% at Farm B). In contrast, soil carbon content in the smaller farms, where fields are on steep slopes, was significantly lower. Overall, larger farms (A and B) tend to have higher soil carbon contents than smaller farms (C and D). The size of organic vegetable farms, combined with the varying topography within each farm, plays a critical role in explaining soil variability.
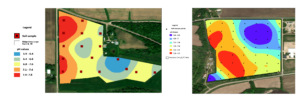
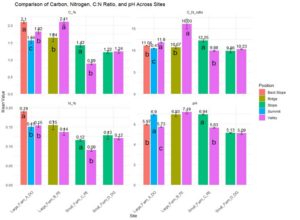
Results in Year 1
We focused our GIS analysis on Featherstone Farm in Year 1 to develop, standardize, and test the methods before applying them to all participating farms. Figure 1 shows the areal distribution of field area by elevation. The graph shows a binomial distribution, indicating that the farm's cultivated fields are located on the valley floor or the ridge.
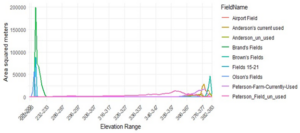
In Figure 2, we analyzed the areal distribution of individual fields by slope gradient. Most fields, except for the currently unused field, have negligible slope gradients. However, the fields differ considerably in terms of within-field variability in slope gradients. For example, Olson's field, Peterson's farm-currently unused, and Brand's field have much greater variations in slope gradients than other fields.
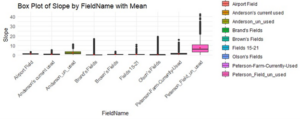
Figure 3 shows the aspect of the fields. Our next step is to convert the aspect of the fields (by 3m X 3m) into solar radiation (energy per area per time). With relatively minimal slope gradients of the fields, the different aspects of the fields may not result in significant differences in solar radiation in this particular farm.
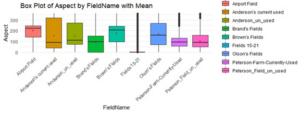
The central question of this project is how topography and soil are related to providing favorable or challenging growing conditions for farmers. For soils, we analyzed the bare ground images to assess the soil brightness index and relate it to soil moisture and organic carbon content. Preliminary analyses show that Brand's field and Peterson's field have the highest brightness index (Figure 4), indicating high moisture and organic matter content. However, these two also have the highest variability (Figure 5).
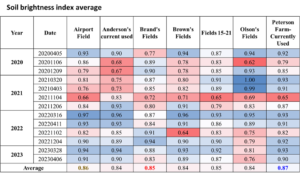
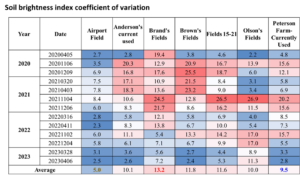
Within this farm, these topographic analyses are beginning to quantitatively reveal the within-field variability of topographic characteristics and solar radiation. They also allow us to identify zones where these characteristics differ significantly from the rest of the fields and require different management.
We also worked with NDVI analyses to characterize the within-field variability. Understanding the NDVI of organic vegetable farms at the field scale proved to be extremely challenging, mainly because of the high spatial and temporal variability of the crops grown in the fields.
Figure 6 shows the seasonal trends of NDVI by different fields, which can potentially be related to crop biomass, but with considerable data collection at the ground level. What could be potentially useful is to characterize the differences in NDVI between fields and the within-field coefficients of variance of NDVI (Figure 7).
Figure 6. NDVI and its seasonal variation by fields.
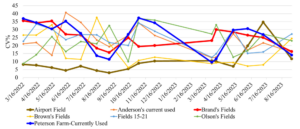
In the light of the above hypotheses, these GIS results show that topography is a critical factor in the management of this farm and that individual fields - depending on their topographic location - exhibit substantially different soil and vegetation characteristics and their spatial and temporal variability.
We also met with the farmers of the four participating organic vegetable farms and visited their farms. The information collected about their fields and farming challenges is described in the project activities. These interviews and field visits confirm that the methods, observations and results developed from Farm B would be applicable to all organic vegetable farms we study in this project, but with important farm- and topography-specific differences.
Based on this progress, we have the following research objectives for the second year:
- Integrate topographic, soil, and vegetation GIS data to divide fields into unique management zones.
- Apply these methods to all participating farms.
- Complete laboratory analyses of soils collected from the Farm D and conduct soil sampling on all participating farms.
- Compare GIS data (e.g., NDVI and bare soil brightness) with ground-based data (crop survey, soil temperature, and soil organic matter analyses).
Education
Our education approach includes (1) direct communication with farmers on the fields, (2) focus group meetings, (3) presentations at organic farming conferences, (4) publishing at farming newsletters, (5) a field day event, and (6) a workshop at organic farming conference.
Project Activities
Educational & Outreach Activities
-Kyungsoo Yoo was invited to present the results from this SARE project at National Institute of Agricultural Sciences in S. Korea (6/24/2024). About 40 agricultural researchers attended the presentation.
Participation Summary:
Year 2
- We presented three research posters at the Marbleseed Organic Farming Conference at La Crosse, Wisconsin (2/20-2/22/2025). We had about 50 farmers and 20 agricultural professionals stopped by the posters.
Junjun Lu, Yuxin, Miao, Azucena Sierra Garcia, Kyungsoo Yoo, Assessing Spatial Variability of Soil Properties, Crop Growth, and Management Using Satellite Remote Sensing in an Organic Farm with Complex Landscapes.
Azucena Sierra Garcia, Kyungsoo Yoo, Julie Grossman, Yuxin Miao, Applied Remote Sensing to Detect Spatial Variability in Sloped Terrains of Vegetable Organic Farming.
Azucena Sierra Garcia, Kyungsoo Yoo, Julie Grossman, Yuxin Miao, Soil Variabilities and Topography in Organic Vegetable Farms in the Driftless Area.
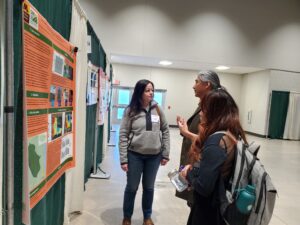
- StoryCorps interviewed us (Kyungsoo Yoo and Azucena Sierra) on our project at the Driftless Area and our academic interests (2/21/2025). The interview is available at:
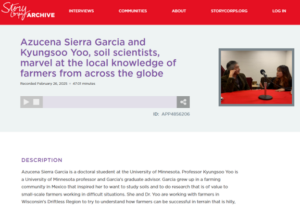
- Graduate student Azucena Sierra introduced our project and shared the results in a workshop organized by Wisconsin Women in Conservation. About 15 farmers, staff, ag coaches, and mentors participated in this workshop and discussed the challenges in farming.
- Kyungsoo Yoo was invited to present the results from this SARE project at National Institute of Agricultural Sciences in S. Korea (6/24/2024). About 40 agricultural researchers attended the presentation.
- We have a lot going on. We are behind the schedule proposed in our proposal. Our proposal scheduled the field day in Year 2 and the workshop in Year 3. In these education and outreach activities, we are 1 year behind. The delay was largely due to the time required to build substantial data to communicate to the farmers. With little published research on organic vegetable farms in the Driftless Area, we had several major trial-and-error in designing field soil sampling and implementing precision ag tools. Now, as we have built experiences in addressing these challenges and collected much of the data, we are beginning to focus on education and outreach activities. To ensure these remaining efforts, we will soon apply to no-cost-extension.
- We met with the leadership in the Featherstone Farm at Rushford, Minnesota. We will collaborate on the field day. The date for the field event is not set yet. We are considering September-October. We have also talked to the Minnesota Organic Conference about the workshop event we plan to hold in early 2026. With the Marbleseed annual conference just completed, we will talk to the organization’s staff to propose our workshop in their next year’s conference.
- We have arranged an article submission to the Organic Broadcaster, a bulletin from the Marbleseed. They expect our article on organic farming in the Driftless Area for their summer issues. The due date for our article is June 6th). We will look for other opportunities to communicate our work and results to organic farmers. Those options include Fruit and Vegetable Newsletter (~1800 subscribers) and Yard and Garden news about gardening on slopes (>8,000 readers). As we now have significant data, we will also publicize soil-related results via the Minnesota Office for Soil Health Twitter account ( >1200 followers, UMN Extension Crop News blog, >3000subscribers).
Year 1.
Through focus group meetings and farm visits, our Year 1 activities focused on identifying farmers' needs and understanding the details of their topographic challenges. An important part of our education and outreach is through these meetings and face-to-face discussions. In addition to these activities, we have also conducted a GIS analysis of individual farms and fields to help address the concerns of the farmers. I have incorporated these activities into research and project activities in this annual report.
We are in the process of writing a journal article on the effects of topography on organic vegetable farms in the Driftless Area. Our findings will also be the basis for a newsletter article in years 2 and 3. In Year 2, we will begin to prepare for the field days and workshops at the meetings. We have contacted and are talking with Marbleseed about co-sponsoring the Field Day.
Learning Outcomes
- Management Area
- Importance of considering topography