Final report for SW17-060
Project Information
Farmers are requesting in-depth knowledge about how to successfully integrate UAS (Unmanned Aerial Systems) into strawberry production to improve management practices. California produces 88% of US strawberries, with an annual value of approximately $2.6 billion, and the California Strawberry Commission identifies spider mite management a key research priority. Little is known about the spatio-temporal dynamics of spider mites in strawberry, and consequently, about the optimal timing to release predatory mites, their natural enemies. In this multi-disciplinary project, we will demonstrate that airborne remote sensing can be used to detect and diagnose spider mite hotspots and therefore pinpoint when and where predatory mite releases are needed. This three-year project will lead to the following outcomes: 1) demonstrate relationships between spider mite presence and unique changes in leaf reflectance features acquired from strawberry plants; 2) a spatially optimized, reliable and practically feasible sampling plan for spider mites in strawberry fields; 3) hyperspectral airborne remote sensing-based characterization of spider mite hotspots to spatially optimize releases of natural enemies. Deployment of novel, labor-extensive, and precise airborne remote sensing technologies to monitor crop health and mitigate pest risks, as well as aerial predator distribution techniques, are highly compatible with existing strawberry management practices. Educational outreach involves lectures on spider mite sampling at grower and ag professional meetings throughout the California strawberry growing region. Growers outside this area will be reached through publications in trade journals and other grower media. Both the educational outreach and research outcomes are easily transferable to strawberry growing regions elsewhere, and could be expanded to include monitoring for other pests and pathogens. This project will enhance efficacy of biocontrol agents and reduce dependence on miticides, enhancing sustainability of spider mite management practices in strawberry.
The research component of this project is based on two fundamental hypotheses (both supported by preliminary data):
1) Spider mite outbreaks are most likely in strawberry plants under abiotic stress (imbalances or deficiencies of nutrient fertilization or inadequate irrigation), and abiotic stress is closely linked to descriptive leaf traits (toughness and micro- and macro-element composition).
2) Airborne remote sensing data of strawberry canopy can be used to detect and map descriptive leaf traits (toughness and micro- and macro-element composition), which are important indicators of risk of spider mite outbreaks.
The educational outreach component of this project is based on the hypotheses that:
1) sampling of strawberry fields can be optimized through development of reliable and practically feasible sampling plans.
2) use of predatory mites for control of spider mite outbreaks in strawberry can be significantly improved through better detection (either through remote sensing or sampling) of spatio-temporal aggregations of emerging spider mite outbreaks.
As part of this 3-year project, we will address the following numbered objectives:
Objective 1: Experimental characterization of the relationship between: 1) leaf micro- and macro-element composition and leaf reflectance, and 2) spider mite-induced stress and leaf reflectance. This has been done in project years 1 (October – December 2017), 2 (January – March 2018), and 3 (February – April and September – November 2019). Quantifiable outcomes: 1) A research article describing a model of the factorial relationships between leaf micro- and macro-element composition, leaf reflectance, and spider mite-induced stress, while varying biotic stressors (i.e., spider mite, beet armyworm, whitefly, and non-infested plants). This because spider mites are rarely the only problematic arthropods in a strawberry field. 2) A research article describing a model of the aforementioned factorial relationships while varying plant fertilizer levels (i.e., 50%, 100%, and 200% fertilizer). This objective has started in October 2017 and has been completed in March 2020. Two replicates were performed for the first experimental study, in project year 1 and project year 2. Two replicates were performed for the second experimental study, both in project year 3. Preliminary results of both studies are described in the section “Results and discussion”.
Objective 2: Characterization of the spatio-temporal distribution of spider mites and their natural enemies in project year 2 (May – September 2018). Quantifiable outcomes: 1) Spatio-temporal data sets from 120 combinations of commercial strawberry field, sampling event, and growing season, 2) A research article describing a spatially optimized sampling plan for spider mites in strawberry fields. Moreover, sampling of spider mites in commercial strawberry fields will provide valuable insight into spatial trends in the distribution of emerging infestations and their spread. That is, we predict to document consistent “edge-effects” of spider mite infestations with highest spider mite densities near strawberry field edges, as edge-biased spatial distributions are commonly observed (Nguyen & Nansen, 2018). Quantitative information about prevailing spatial distribution patterns is critically important as part of optimizing both sampling/detection and releases of natural enemies. This objective has started in May 2018, and was completed in March 2020. We have collected over 1,500 strawberry samples on 4 commercial farms, both managed conventionally and organically. We are currently analyzing the data, and preliminary results are described in the section “Results and discussion”.
Objective 3: Hyperspectral airborne remote sensing-based characterization of hotspots with emerging spider mite outbreaks in project years 1 (May – July 2017) and 2 (May – July 2018). Quantifiable outcomes: 1) Hyperspectral airborne remote sensing data sets from 120 combinations of commercial strawberry field, sampling event, and growing season, 2) A research article describing the use of hyperspectral airborne remote sensing to spatially optimize releases of natural enemies to control spider mite outbreaks in strawberry fields, 3) A review article summarizing the available literature on use of drones in sustainable agriculture (published in December 2019). This objective has been completed in March 2020. Preliminary results are described in the section “Results and discussion”.
Objective 4: Educational outreach on how to improve spider mite sampling and on the potential of UAS in strawberry management and beyond. Quantifiable outcomes: 1) Dissemination of project results to a minimum of 300 growers and other stakeholders. 2) Publication of a review paper on use of UAS in sustainable agriculture; the paper will be distributed to the California Strawberry Commission and other stakeholder organizations. This objective has been completed in March 2020.
Cooperators
- (Researcher)
- (Researcher)
- (Researcher)
- (Researcher)
- (Researcher)
- (Researcher)
- (Researcher)
- (Researcher)
Research
Objective 1. We hypothesize that strawberry canopy reflectance features vary significantly and uniquely in response to nutrient deficiencies and spider mite infestations. Most previous studies involve only one stressor. However, in a realistic situation, multiple stressors, such as, nutrient deficiencies and arthropod pests, will be present in commercial crop fields. In order to optimize pest management and control, using remote sensing, we aim to distinguish strawberry infestation with spider mites from a) strawberry infestations with other types of pests, and b) abiotic stress, such as, nutrient deficiencies.
Objective 2. We hypothesize that, in a commercial field, spider mites are not equally distributed over the field, but aggregate in suitable spots for their growth and reproduction, such as, at the field edge (i.e., the edge-effect). To optimize detection and sampling methods, as well as proper management (i.e., release of natural enemies), we aim to characterize spider mite distribution patterns over space and time.
Objective 3. We hypothesize that, in a commercial field, strawberry plant reflectance features vary significantly when attacked by spider mites and when healthy. In order to optimize pest management and control, we aim to characterize spider mite hotspots based on airborne remote sensing.
Objective 1. A. We hypothesize that strawberry canopy reflectance features change significantly and uniquely in response to specific biotic stressors, such as spider mites. This experiment was first performed in project year 1, and repeated in project year 2. To characterize the “uniqueness” of reflectance responses by strawberry plants to spider mites, we conducted an experimental greenhouse study, in which individually potted strawberry plants were divided into four treatments: 1) non-infested control plants, 2) plants infested with spider mites, 3) plants infested with whiteflies, and 4) plants infested with beet armyworms (Figure 5a, Figure 6c-e). These different biotic stressors were chosen as they represent important pests of California strawberry with different feeding biology (UC IPM, 2020). Plants were individually kept in screen cages (BugDorm-2120F insect rearing tents, BioQuip Products, Rancho Dominguez, CA).We acquired data on leaf reflectance from the plants at 0 (baseline), 1, 3, 7, 10 and 14 days after infestation (DAI). We acquired data on photosynthesis, leaf element composition, and biomass from the plants after 0 (baseline) and 14 DAI.
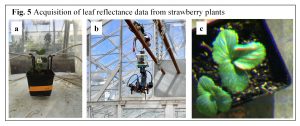
Figure 6: Assessing strawberry leaf reflectance in response to different biotic stressors. (a) The experimental set-up: strawberry plants lined up under a hyperspectral camera mounted on a rail system inside a greenhouse (photographer: Alison N. Stewart). (b) Hyperspectral image, or hypercube (three-dimensional dataset), of a strawberry plant. (c) Two-spotted spider mite. (d) Beet armyworm. (e) Silverleaf whitefly (photographer: Ian M. Wright).
Hyperspectral imaging data were acquired using a true push-broom hyperspectral camera with 116 spectral bands from 470-980nm (OCI Imager, OCI-UAV-D1000, BaySpec, San Jose, CA), which was mounted on a rail system inside a greenhouse (Figure 5b, Figure 6a). However, to eliminate spectral bands associated with high signal/noise ratio, we only included data in 106 spectral bands from 513-967 nm in the analyses. Figures 5c and 6b show the actual spatial resolution of the acquired hyperspectral imaging data.
A grounded hyperspectral spectrometer (SuperGamut VIS-NIR [visible and near-infrared], Bayspec, imaging spectrometer, 380-1020 nm wavelength range, here referred to as the “reference spectrometer”) was mounted in vertical position and with the lens 50 cm above a piece of matte white Teflon (referred to as white calibration board). Data from the reference spectrometer is used for continuous white calibration of leaf reflectance data. This component is one of the most unique and important aspects of this project, because it enables high-precision calibration of leaf reflectance data, and therefore markedly higher likelihood of accurately detecting subtle reflectance responses to biotic stressors.
Data on photosynthesis, as well as the related factors stomatal conductance, transpiration rate, and intercellular CO2, were obtained with a LI-6400XT Portable Photosynthesis System (LI-COR Biosciences, Lincoln, NE). Measurements were taken from the youngest fully expanded leaves. Also, we obtained plant aboveground fresh weight. All plants were inspected under a microscope to verify the presence of arthropod infestations on infested plants (spider mites and whiteflies were counted and beet armyworm damage could be observed by eye), and the absence of infestations on control, non-infested plants. Afterwards, plant material was dried in a drying room at 55°C for seven days, and dry weight measurements were taken. Dry leaf material was ground to a fine powder and shipped to a commercial laboratory (A&L Great Lakes Laboratories, Fort Wayne, IN) for element composition analysis (N, S, P, K, Mg, Ca, Na, B, Zn, Mn, Fe, Cu, and Al).
Leaf reflectance data from 0 and 14 DAI (replicate 1) were subjected to advanced data processing and filtering and we then classified data based on the following parameters: 1) all leaf reflectance data from 0 DAI were considered “control” (non-infested), and 2) leaf reflectance data from 14 DAI were assigned to their respective treatments. Details on processing and classification of reflectance data have been described extensively in studies published by co-PI Nansen (Nansen, 2011; 2012; Nansen & Elliott, 2016; Nansen et al., 2013a; Nguyen & Nansen 2020; Nguyen et al., 2020; Qi et al., 2011; Zhao et al., 2013; Zhao et al., 2014).
B. We hypothesize that strawberry canopy reflectance features change significantly and uniquely in response to a combination of specific biotic stressors (spider mites) and abiotic stressors (nutrient deficiencies). This experiment was performed and then repeated in project year 3. To characterize the “uniqueness” of reflectance responses by strawberry plants to spider mites and different fertilizer levels, we conducted an experimental greenhouse study, in which individually potted strawberry plants received three fertilizer treatments: 1) 50%, 2) 100%, and 3) 200% fertilizer (Figure 12ab). Plants subjected to each fertilizer treatment were divided into two groups, and subjected to the following arthropod treatments: 1) control, non-infested plants, 2) plants infested with spider mites (Figure 12c). Thus, strawberry plants were divided into six treatments: 1) control, 50% fertilizer, 2) spider mites, 50% fertilizer, 3) control plants, 100% fertilizer, 4) spider mites, 50% fertilizer, 5) control, 200% fertilizer, 6) spider mites, 200% fertilizer (Figure 12g). We established the optimal fertilizer rate for strawberry (100%) based on published literature (Suarez et al., 2013; Lee et al., 2015; Sun et al., 2015; Gonzalez-Fuentes et al., 2016) and communication with other strawberry researchers at UC Davis. Based on this value, we provided plants with a nutrient deficiency (50%) and a surplus (200%). Plants were individually kept in screen cages (BugDorm-2120F insect rearing tents, BioQuip Products, Rancho Dominguez, CA). They received the different fertilizer treatments from the moment of planting. After approximately 5 weeks, half of the plants that received each fertilizer treatment were infested with two-spotted spider mite, and half of the plants remained uninfested. We acquired data on leaf reflectance from the plants before infestation (baseline), and 1, 2, and 3 weeks after infestation (Figure 12 d-f). We acquired data on photosynthesis, leaf element composition, and fresh and dry biomass from the plants before infestation (baseline) and 3 weeks after infestation.
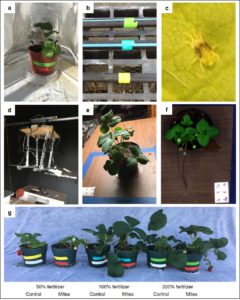
Hyperspectral imaging data were acquired using a Pika L VNIR (visible and near-infrared) hyperspectral imaging camera with 281 spectral bands from 400-1000nm (Pika L, Resonon, Bozeman, MT), which was mounted on a rail system inside a darkened room (Figure 12d). It should be noted that this set-up was different from the one used in previous years to assess strawberry canopy reflectance features in response to multiple biotic stressors (section “A” above). First and foremost, we purchased a novel, state-of-the-art hyperspectral sensor (independent of Western SARE funds). While our previous sensor, the OCI Imager, was the best product on the market when purchased, recent developments resulted in improved technology. The Pika L provides two major improvements compared to the OCI Imager: 1) it has a broader spectral range, and 2) one sensor contains this entire spectral range (the OCI Imager has one sensor for the visible and one for the near-infrared range). Thus, the Pika L eliminates the time-consuming and error-inducing step of overlaying the images from two sensors before data analysis. The Pika L arrived in January 2019, and after a short period of training with the camera and related software, we started taking measurements in March 2019. Second, we developed a novel rail system in a dedicated darkened room. Previously, we obtained images in a greenhouse during daytime, but we experienced interference from sunlight. Obtaining data in a darkened room, with the rail system’s own lights as the sole light source, allows for more reliable data collections. Data on photosynthesis and biomass were obtained as described above for section A. Dry leaf material was ground to a fine powder and element composition analysis (N, S, P, K, Mg, Ca, Na, B, Zn, Mn, Fe, and Cu) was performed at the UC Davis Analytical Lab. All plants were inspected under a microscope to verify the presence of arthropod infestations on infested plants (we counted spider mite eggs and mobiles (nymphs + adults)), and the absence of infestations on control, non-infested plants.
Objective 2. We hypothesize that spider mites occur in high densities near strawberry field edges, i.e. the edge-effect. To address this hypothesis, we selected spider mite-infested strawberry fields (information obtained from the growers), where we collected leaf samples from 0.5-1.5 acre plots. A total of 92-100 samples were collected each week for a total of three consecutive weeks (Table 2). Samples were collected haphazardly, starting at a field edge and walking forth and back among rows. The exact site of each sample was recorded with a handheld GPS, the GPSMAP 64st (Garmin, Olathe, KS). Samples, one trifoliate strawberry leaf, were collected in pre-labeled Ziploc bags, stored in a mesh bags for the duration of sampling, stored in a cooler for the duration of travel, and stored in a freezer at -20°C until analysis (Figure 7). In the laboratory, under a microscope, we counted the number of eggs, nymphs, males, and females of two-spotted spider mite and Lewis mite, both important pests of California strawberry (Dara, 2011, 2014a). Also, we counted the number of Phytoseiulus persimilis and Neoseiulus californicus, important commercially available natural enemies of spider mites in California strawberry (Dara, 2014b). Spider mite numbers (two-spotted spider mite and Lewis mite combined) for the first sampling week of a number of sites have been reported.
Spider mite numbers were spatially plotted using the “ggplot” function in the “ggplot2” package in R (version 3.5.0). Moran’s I was calculated using the “Moran.I” function in the “ape” package. Moran’s I is a measure for spatial autocorrelation, or, the expectation that two measurements at nearby locations are more closely related than two measurements at locations further apart (Fortin et al., 2013). Ia, the index of aggregation, was calculated using the “sadie” function in the “epiphy” package. SADIE (Spatial Analysis by Distance IndicEs) aids in the spatial analysis of ecological count data. When Ia < 1, spider mite distribution patterns follow a regular distribution. When Ia = 1, this indicates a random distribution. When Ia > 1, this indicates an aggregated distribution (Campos-Herrera et al., 2013). Co-PI Nansen has extensive experience in spatial data analysis with SADIE (Martini et al., 2012; Nansen et al., 2004; Severtson et al., 2015; Weaver et al., 2005).
Objective 3. In project year 1, we identified pairs of commercial strawberry fields in the same strawberry growing area, of which one was healthy, and one was heavily infested with spider mites. It is important that the strawberry variety in both fields is the same, as our hyperspectral camera is sensitive enough to detect differences between varieties. We used the same hyperspectral camera and continuous white calibration as described under project results for objective 1 A. We developed a classification method to distinguish spider mite-infested from healthy strawberry plants, and we used the same processing and classification procedures as described extensively in studies published by co-PI Nansen (Nansen, 2011; 2012; Nansen & Elliott, 2016; Nansen et al., 2013a; Nguyen & Nansen 2020; Nguyen et al., 2020; Qi et al., 2011; Zhao et al., 2013; Zhao et al., 2014). Technical difficulties with both the drone and the hyperspectral camera limited data collections.
In project year 2, we marked strawberry fields with cones, where we collected samples as described above for objective 2 (Figure 8a). In this way, we identified healthy and spider mite-infested plants in individual strawberry fields, reducing the possibility that different growing conditions could confound the data. The cones were clearly visible in the hyperspectral images (Figure 8b). Spider mite numbers were spatially plotted as described above for objective 2.
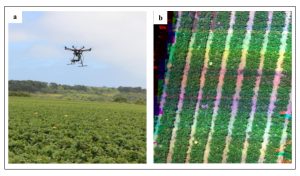
In project years 1 and 2, PI De Lange wrote a review article on the various uses of drones in sustainable pest management. This was in collaboration with Fernando Iost-Filho, MSc, graduate student in drone-based remote sensing at the University of Sao Paulo, Brazil; Dr. Wieke Heldens, remote sensing expert at the German Aerospace Center (DLR), Germany; and Dr. Zhaodan Kong, assistant professor in the Department of Mechanical and Aerospace Engineering at UC Davis, expert in drone-drone communication. The paper was submitted in project year 3, to Journal of Economic Entomology, a leading entomological journal.
Objective 1. A. We acquired data on leaf reflectance from strawberry plants in individual pots after 0 (baseline), 1, 3, 7, 10 and 14 days of infestation (DAI) with spider mites, whiteflies, and beet armyworm, as well as control, non-infested plants. We acquired data on photosynthesis, leaf element composition, and biomass from the plants after 0 (baseline) and 14 DAI. We repeated the experiment twice, with similar results. Here, we present hyperspectral imaging data from 0 and 14 DAI (replicate 1), and nutrient data from 14 DAI (both replicate 1 and replicate 2).
In the first replicate, at 14 DAI, spider mite-infested plants contained 159 ± 75.2 eggs (average ± standard error) and 193 ± 52.3 nymphs and adults (mobiles). Whitefly-infested plants contained, on average, 27 ± 7.5 eggs and 26 ± 8.1 nymphs. In addition, it was confirmed that strawberry plants infested with beet armyworms (3 second-instar larvae) all experienced extensive damage. Thus, arthropod infestations were successful under the experimental conditions.
In the second replicate, arthropod infestations were also successful under the experimental conditions. Spider mite-infested plants contained 51 ± 19.5 eggs and 195 ± 27.2 nymphs and adults (mobiles). Whitefly-infested plants contained, on average, 41 ± 22.1 eggs and 15 ± 5.6 nymphs. Beet armyworms caused extensive damage.
In the first replicate, for three of the leaf elements (Mg, K, and P), there was a significant effect of biotic stress at 14 DAI (P < 0.05), while the remaining ten leaf elements (N, S, Ca, Na, B, Zn, Mn, Fe, Cu, and Al) did not show a significant response to biotic stressors (P > 0.05). Moreover, we found that significant differences in levels of Mg (Figure 1a), K (Figure 1b) and P (Figure 1c), were attributed to differences between plants subjected to beet armyworms and spider mites (different letters above bars indicate statistically significant differences). We observed a negative association between spider mite infestation and low potassium leaf content, which is consistent with published research by co-PI Nansen (Nansen et al., 2013b; West & Nansen, 2014). In addition, published research by co-PI Nansen has shown that low potassium leaf content has been associated with higher likelihood of infestation by two aphid species (Lacoste et al., 2015; Severtson et al., 2016). Thus, we have provided initial evidence of strawberry plants showing partial element composition responses to different biotic stressors.
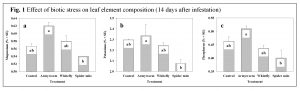
In the second replicate, for two of the leaf elements, N (Figure 9a) and P (Figure 9b), there was a significant effect of biotic stress at 14 DAI (P < 0.05). N can have a major impact on pest resistance (Altieri & Nicholls, 2003), and differential P levels were also identified in replicate 1. However, in replicate 2, we did not find increases or reductions of specific elements associated with spider mite infestation. We will combine the element data from both replicates for analysis in further detail, to elucidate potential differences in element patterns in response to the different biotic stressors.
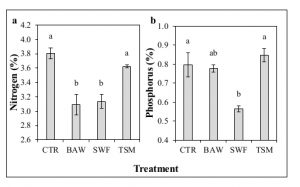
In the first replicate, average reflectance profiles from strawberry plants at 0 DAI (Figure 2a) showed that there was negligible difference in leaf reflectance prior to exposure to biotic stressors. Figure 2b shows that at 14 DAI, especially beet armyworms and spider mites caused considerable increase in leaf reflectance. Figure 2c shows the same data as presented in Figure 2a and 2b, except that the 14 DAI data were divided with the average reflectance profiles from 0 DAI. Thus, Figure 2c shows the relative change over time in terms of average leaf reflectance, with: 1) values >1 indicating an increase in reflectance, 2) values < 1 indicating a decrease in reflectance, and values close to 1 indicating little or no change in reflectance. From Figure 2c, it is seen that: 1) non-infested control plants had the least change in reflectance within the 14-day time period, 2) whitefly infestation appeared to have negligible effect on leaf reflectance (very similar average reflectance values as non-infested control plants), 3) beet armyworm infestation caused a marked increase in leaf reflectance, especially at 648 nm, and 4) spider mite infestation caused a modest increase in leaf reflectance in spectral bands from 580-665 nm and a similar response as beet armyworm infestation in spectral bands from 750-980 nm.
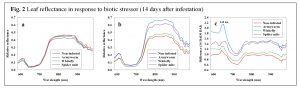
It is important to emphasize that the hyperspectral imaging data were acquired inside a greenhouse, which means that glass interfered with the obtained leaf reflectance data. It is therefore not possible to directly translate the obtained imaging data into commercial field conditions. However, the data are very important and encouraging as they demonstrate that it may be feasible to uniquely separate spider mite infestations from other biotic stressors of strawberry plants.
A total of 156 average reflectance profiles from potted strawberry plants were analyzed, and the results from the classification are presented in a confusion matrix (Table 1). In Table 1, the left column lists the actual treatments and the other columns lists how the average reflectance profiles from the different treatments were classified. Thus, all (100%) of average reflectance profiles from armyworm infested strawberry plants were classified as armyworm infested. In addition, 80% of average reflectance profiles from spider mite infested strawberry plants were classified as spider mite infested (5% were incorrectly classified as armyworm infested, and 5% were incorrectly classified as whitefly infested). Across the four treatments, the leaf reflectance based classification of plants was 85%, which is well within the acceptable accuracy range for these types of applications of reflectance based analyses.
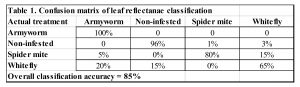
B. We acquired data on leaf reflectance from strawberry plants in individual pots exposed to 50%, 100%, or 200% fertilizer before infestation (baseline), and 1, 2, and 3 weeks after infestation with spider mites. We acquired data on photosynthesis, leaf element composition, and biomass from the plants before infestation (baseline) and 3 weeks after infestation. We repeated the experiment twice, with similar results. Here, we present data on plant fresh weight for both replicate 1 and 2, and the number of eggs and mobiles on plants for replicate 1. Analysis of hyperspectral imaging, photosynthesis, and nutrient data for all timepoints for both replicates is still in progress.
For both replicate 1 (Figure 13a) and replicate 2 (Figure 13b), strawberry plants that received 100% or 200% fertilizer obtained a higher biomass than plants that received 50% fertilizer, irrespective of spider mite infestation status. Thus, a nutrient deficiency decreased plant biomass, while a nutrient surplus did not affect plant biomass.
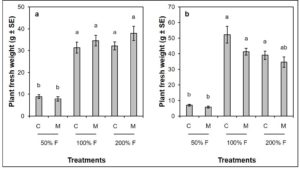
For replicate 1, spider mites deposited a higher number of eggs on strawberry plants that received 100% or 200% fertilizer compared to plants that received 50% fertilizer. Thus, it seems that a nutrient deficiency negatively impacts spider mite fecundity (Figure 14a). There were no significant differences in the number of mobile spider mites on plants that received each fertilizer treatment (Figure 14b). Overall, levels of individual elements were higher on plants that received 100% and 200% fertilizer than on plants that received 50% fertilizer, indicating that while supplying 50% fertilizer resulted in a nutrient deficiency, supplying 200% fertilizer did not notably affect plant nutrient content. The presence of spider mites did not notably affect plant nutrient content (Figure 15ab). Analysis of hyperspectral imaging data is still in progress.
Objective 2. We collected strawberry leaf samples to characterize the spatio-temporal distribution of spider mites. Preliminary results show random patterns of spider mite (eggs + mobiles) distribution in four out of five strawberry fields in the Santa Maria and Oxnard area, i.e., no indication of an edge-effect (Ia values ranging from 0.86 to 1.27, P > 0.05, Table 3, Figure 10abce). In one of the fields, we observed an aggregated distribution of spider mites (Ia = 1.59, P < 0.05, Table 3, Figure 10d). No autocorrelation was observed in any of the fields, i.e., spider mite numbers on nearby plants were not more similar than numbers on plants further away (Table 3). These results may eventually contribute to targeted sampling efforts, focusing on areas in strawberry fields where spider mites are most likely to occur.
When analyzing the data from later timepoints, we decided to plot only mobile spider mites, as large egg batches could confound the results. These results show random patterns of spider mite distribution in all sampled strawberry fields (Table 4, Figure 16). Autocorrelation was observed in one of the fields, only at one timepoint (Table 4). These results will shed more light on the distribution of important herbivores and predators in strawberry fields.
Objective 3. As part of this project, we intend to demonstrate that airborne remote sensing can be used to detect hotspots with emerging spider mite outbreaks based on unique reflectance responses by strawberry plants to spider mite infestations. In project year 1, we conducted flight missions above non-infested strawberry fields and above hotspots with emerging spider mite outbreaks (Figure 3a). Flying at an altitude of 30 m, we obtained hyperspectral imaging data as shown in Figure 3b, in which the multiple pixels are acquired from individual strawberry leaves. As part of the data analysis, we established two categories of “soil” (with or without shadow), and representative pixels were selected from hyperspectral images of non-infested and infested hotspots. Figure 3c shows the average reflectance profiles from the four categories (soil with or without shadow and non-infested and infested hotspots). In addition, the black curve indicates the difference between infested and non-infested strawberry plants (infested / non-infested), and it is seen that spider mite infestation causes a marked increase in leaf reflectance in spectral bands from 700-980 nm. In a study of spider mite infestations of maize plants, co-PI Nansen found very similar results; a spectral band near 740 nm was particularly indicative of spider mite infestation (Nansen et al., 2013b).
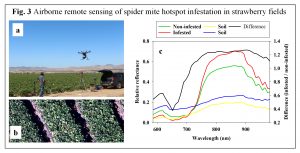
As an example of how the airborne hyperspectral data may be used, Figure 4 shows two locations without (Figure 4a) and with (Figure 4b) ground-based confirmation of spider mite infestation of strawberry plants. The maps of hotspots were generated on the basis of processed and classified hyperspectral imaging data, and the maps clearly indicate that airborne remote sensing data may be able to detect spider mite infestations. We wish to point out that at the infested hotspot, 5-10 spider mites were found on most leaves, so this represented an unusually high infestation level. Considerably more field data during growing seasons and among growers are needed before we can comfortably argue that spider mite infestation hotspots can be detected consistently and with sufficient accuracy. However, the preliminary data are encouraging, and the field data are partially supported by the reflectance responses shown in the experimental greenhouse study (Figure 2).
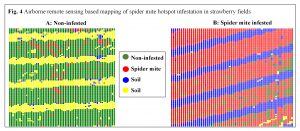
In project year 2, we improved our experimental set-up, and conducted flight missions above strawberry fields where sections were marked with colored cones. Plant material was collected form these marked spots, and examined for the presence of spider mite eggs and mobiles, in order to plot the approximate density and distribution of spider mites. An example of the resulting plot, displaying potential spider mite hotspots, can be seen in Figure 11. These types of plots help us decide on which sections of the corresponding hyperspectral image, obtained with the drone, to focus when assessing spider mite-infested and healthy plants in the strawberry fields.
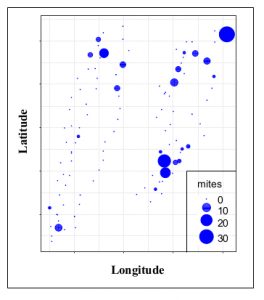
In project year 3, we published a review paper on the various uses of drones in sustainable pest management, in the Journal of Economic Entomology (Iost Filho et al., 2020). This journal has an impact factor of 1.779 and is among the most-cited entomological journals, thus ensuring a large, relevant scientific audience. The paper, which contains an extensive literature review on drones in agriculture, will form the basis of the research papers resulting from this project that are currently in progress. Also, the paper may inspire other researchers to use drones to address various agriculturally relevant research questions. Drone-based remote sensing could be an important tool alongside other methods, such as selection of resistant varieties and use of biocontrol organisms, contributing to sustainable, integrated pest management (IPM) in strawberry and other crops (Dara, 2019, 2020).
References Cited
Altieri MA & Nicholls CI (2003) Soil fertility management and insect pests: Harmonizing soil and plant health in agroecosystems. Soil and Tillage Research 72: 203-211.
Campos-Herrera R, Ali J, Diaz B & Duncan L (2013) Analyzing spatial patterns linked to the ecology of herbivores and their natural enemies in the soil. Frontiers in Plant Science 4: 378.
Dara SK (2011) Twospotted spider mite and Lewis mite: a comparison. E-Journal of Entomology and Biologicals. https://ucanr.edu/blogs/blogcore/postdetail.cfm?postnum=5771
Dara SK (2014a) Managing spider mites in California Strawberries. E-Journal of Entomology and Biologicals. https://ucanr.edu/blogs/blogcore/postdetail.cfm?postnum=13943
Dara SK (2014b) Predatory mites for managing spider mites on strawberries. E-Journal of Entomology and Biologicals. https://ucanr.edu/blogs/strawberries-vegetables/index.cfm?tagname=Predatory%20mites%20for%20spider%20mite%20management
Dara SK (2019) The new integrated pest management paradigm for the modern age. Journal of Integrated Pest Management 10: 12.
Dara SK (2020) Implementation of IPDM in strawberries and other berries. In Gullino ML, Albajes R & Nicot PC (eds), Integrated Pest and Disease Management in Greenhouse Crops. Springer, Basel, Switzerland, pp. 597-624.
Fortin M-J, Dale MRT & Ver Hoef JM (2013) Spatial analysis in ecology. In El-Shaarawi AH & Piegorsch WW (eds), Encyclopedia of Environmetrics, 2nd edition. John Wiley & Sons, Ltd., Hoboken, NJ.
Gonzalez-Fuentes JA, Shackel K, Lieth JH, Albornoz F, Benavides-Mendoza A & Evans RY (2016) Diurnal root zone temperature variations affect strawberry water relations, growth, and fruit quality. Scientia Horticulturae 203: 169-177.
Iost Filho FH, Heldens WB, Kong Z & De Lange ES (2020) Drones: innovative technology for use in precision pest management. Journal of Economic Entomology 113: 1-25.
Lacoste C, Nansen C, Thompson S, Moir-Barnetson L, Mian A, McNee M & Flower KC (2015) Increased susceptibility to aphids of flowering wheat plants exposed to low temperatures. Environmental Entomology 44: 610-618.
Lee YH, Yoon, CS, Park, NI & Yeoung YR (2015) Influence of various nutrient concentraitons on the growth and yield of summer strawberry cultivars cultivated in a hydroponic system. Horticulture, Enviroment, and Biotechnology 56: 421-426.
Martini X, Seibert S, Prager SM & Nansen C (2012) Sampling and interpretation of psyllid nymph counts in potatoes. Entomologia Experimentalis et Applicata 143: 103-110.
Nansen C (2011) Robustness of analyses of imaging data. Optics Express 19: 15173-15180.
Nansen C (2012) Use of variogram parameters in analysis of hyperspectral imaging data acquired from dual-stressed crop leaves. Remote Sensing 4: 180-193.
Nansen C & Elliott NC (2016) Remote sensing and reflectance profiling in entomology. Annual Review of Entomology 61: 139-158.
Nansen C, Geremias LD, Xue Y, Huang F & Parra JR (2013a) Agricultural case studies of classification accuracy, spectral resolution, and model over-fitting. Applied Spectroscopy 67: 1332-1338.
Nansen C, Sidumo AJ, Martini X, Stefanova K & Roberts JD (2013b) Reflectance-based assessment of spider mite “bio-response” to maize leaves and plant potassium content in different irrigation regimes. Computers and Electronics in Agriculture 97: 21-26.
Nansen C, Subramanyam B & Roesli R (2004) Characterizing spatial distribution of trap captures of beetles in retail pet stores using SADIE® software. Journal of Stored Products Research 40: 471-483.
Nguyen HDD & Nansen C (2018) Edge-biased distributions of insects. A review. Agronomy for Sustainable Development 38: 11.
Nguyen HDD & Nansen C (2020) Hyperspectral remote sensing to detect leafminer-induced stress in bok choy and spinach according to fertilizer regime and timing. Pest Management Science 76: 2208-2216.
Nguyen HDD, Pan V, Pham C, Valdez R, Doan K & Nansen C (2020) Night-based hyperspectral imaging to study association of horticultural crop leaf reflectance and nutrient status. Computers and Electronics in Agriculture 173: 105458.
Qi B, Zhao C, Youn E & Nansen C (2011) Use of weighting algorithms to improve traditional support vector machine based classifications of reflectance data. Optics Express 19: 26816-26826.
Severtson D, Callow N, Flower K, Neuhaus A, Olejnik M & Nansen C (2016) Unmanned aerial vehicle canopy reflectance data detects potassium deficiency and green peach aphid susceptibility in canola. Precision Agriculture 17: 659-677.
Severtson D, Flower K & Nansen C (2015) Nonrandom distribution of cabbage aphids (Hemiptera: Aphididae) in dryland canola (Brassicales: Brassicaceae). Environmental Entomology 44: 767-779.
Suarez DL & Grieve CM (2013) Growth, yield, and ion relations of strawberry in response to irrigation with chloride-dominated waters. Journal of Plant Nutrition 36: 1963-1981.
Sun Y, Niu G, Wallace R, Masabni J & Gu M (2015) Relative salt tolerance of seven strawberry cultivars. Horticulturae 1: 27-43.
UC IPM (2020) Agriculture: Pest Management Guidelines – Strawberry. https://www2.ipm.ucanr.edu/agriculture/strawberry/
Weaver DK, Nansen C, Runyon JB, Sing SE & Morrill WL (2005) Spatial distributions of Cephus cinctus Norton (Hymenoptera: Cephidae) and its braconid parasitoids in Montana wheat fields. Biological Control 34: 1-11.
West K & Nansen C (2014) Smart-use of fertilizers to manage spider mites (Acari: Tetrachynidae) and other arthropod pests. Plant Science Today 1: 161-164.
Zhao C, Qi B & Nansen C (2013) Use of local fuzzy variance to extract the scattered regions of spatial stray light influence in hyperspectral images. Optik 124: 6696-6699.
Zhao C, Qi B, Youn E, Yin G & Nansen C (2014) Use of neighborhood unhomogeneity to detect the edge of hyperspectral spatial stray light region. Optik 125: 3009-3012.
Use of drones has enormous potential for sustainable pest management. Sensing drones, equipped with remote sensing technology, could contribute to faster and more reliable detection of arthropod pests in agricultural fields. Actuation drones, equipped with pesticide spray rigs or dispensers for biocontrol organisms, could deliver solutions to the precise areas where these are needed (Iost Filho et al., 2020).
Our greenhouse studies indicate that it may be feasible to uniquely separate spider mite infestations from other biotic stressors of strawberry plants based on remote sensing technology. This may be associated with changes in leaf element composition in response to various biotic stressors (spider mites, whitefly, and armyworm). Spider mites are among the most important pests of California strawberry, and they are worldwide pests of a large number of field and greenhouse crops. They reproduce very fast, and they are resistant to the majority of available pesticides. The ability to accurately detect spider mite problems could lead to a timelier response, leading to more effective and sustainable control.
Our field studies indicate that spider mites distribution in strawberry fields is random and not aggregated. However, the existence of spider mite hotspots is frequently reported. Likely, spider mite infestations spread quickly after initial presence in specific hotspots, stressing the need for accelerated detection and control.
Our field studies further indicate that drone-based remote sensing technology could accurately detect spider mite outbreaks in commercial strawberry fields. Leaf reflectance profiles were notably different between plants in non-infested fields and in spider mite-infested fields.
Currently, technical issues with the drone and the time-consuming nature of analyzing hyperspectral imaging data limit use of this technology for individual growers. However, consulting companies offer drone-based remote sensing services for a reasonable fee, and imminent technological advances will make the technology more readily available. Thus, drone-based remote sensing could be an important tool alongside other methods, such as selection of resistant varieties and use of biocontrol organisms, contributing to sustainable IPM practices in strawberry and other crops (Dara, 2019, 2020).
Research Outcomes
Education and Outreach
Participation Summary:
Journal articles, peer-reviewed:
Iost Filho FH, Heldens WB, Kong Z, De Lange ES (2020) Drones: innovative technology for use in precision pest management. Journal of Economic Entomology 113: 1-25.
Journal articles, conference abstracts:
De Lange ES, Nansen C (2019) Early detection of arthropod-induced stress in strawberry using innovative remote sensing technology. Front. Vet. Sci. Conference Abstract: GeoVet 2019. Novel spatio-temporal approaches in the era of Big Data. http://dx.doi.org/10.3389/conf.fvets.2019.05.00104
Past presentations:
Elvira de Lange: Poster presentation at the Entomological Society of America (ESA) International Branch Virtual Symposium. Audience: Researchers and industry professionals. Title: Diagnosing spider mite-induced stress in strawberry using airborne remote sensing technology. April 27-29, 2020.
Elvira de Lange: Oral presentation at the Virtual Ecology and Evolution Conference. Audience: Researchers. Title: Drones: emerging technology for sustainable pest management. April 25, 2020.
Christian Nansen: Oral presentation at the Ontario Berry Growers Meeting, Niagara Falls, Ontario, Canada. Audience: Growers, industry professionals, and researchers. Title: Leaf reflectance responses by strawberry plants to fertilizer regimes and arthropod stressors. February 18, 2020.
Christian Nansen: Oral presentation at the Ontario Fruit and Vegetable Convention, Niagara Falls, Ontario, Canada. Audience: Growers, industry professionals, and researchers. Title: Optimization of drone-based releases of predatory mites to control spider mites in strawberry fields – cutting-edge research. February 19, 2020.
Elvira de Lange: Oral presentation at GeoVet, Davis, CA, USA. Audience: Researchers and industry professionals. Title: Early detection of arthropod-induced stress in strawberry using innovative remote sensing technology. October 8-10, 2019.
Elvira de Lange: Oral presentation at the Festival of Fruit, the annual meeting of the California Rare Fruit Growers, Arcadia, CA, USA. Audience: Rare exotic fruit enthusiasts, hobbyists and amateur horticulturists. Title: Beating the bugs: sustainable pest management in strawberry. August 17, 2019.
Elvira de Lange: Oral presentation at the monthly meeting of the California Rare Fruit Growers, Foothill Chapter, Arcadia, CA, USA. Audience: Rare exotic fruit enthusiasts, hobbyists and amateur horticulturists. Title: Drones, apps, and lures for integrated pest management in small fruits. April 6, 2019.
Christian Nansen: Oral presentation at the 9th North American Strawberry Symposium, Orlando, FL, USA. Audience: Strawberry growers, pest control advisers, industry professionals, and researchers. Title: Aerial remote sensing and its potential regarding detection of spider mite outbreaks in strawberry fields and delivery of beneficials. February 3-6, 2019.
Christian Nansen: Oral presentation at Biocontrols USA East, Rochester, NY, USA. Audience: Researchers and industry Professionals. Title: Machine vision, drones, and natural enemies: the next step in protecting your crop. October 11-12, 2018.
Christian Nansen: Oral presentation at the Canadian Greenhouse Conference, Niagara Falls, Ontario, Canada. Audience: Researchers and industry professionals. Title: Use of imaging technologies to detect and diagnose insect stressors. October 3-4, 2018.
Christian Nansen: Oral presentation at the International Conference on Green Plant Protection Innovation, Hainan, China. Audience: Researchers and industry professionals. Title: Use of machine vision technologies to detect and diagnose biotic plant stressors. May 9-12, 2018.
Elvira de Lange: Seminar at San Jose State University, San Jose, CA, USA. Audience: Researchers and undergraduate students. Title: Sprays, lures and drones for sustainable pest management. November 7, 2018.
Elvira de Lange: Seminar at Texas A&M University, College Station, TX, USA. Audience: Researchers. Title: Drone-guided releases of predatory mites for management of spider mites in strawberry. January 31, 2018.
Elvira de Lange: Oral presentation at the Western Alfalfa Seed Growers Association, 49th Annual Winter Seed Conference, San Antonio, TX, USA. Audience: Alfalfa growers, pest control advisers, industry professionals, and researchers. Title: Unmanned Aerial System (UAS)-guided releases of predatory mites for management of spider mites in strawberry. January 28-30, 2018.
Christian Nansen: Oral presentation at Plantekongres 2018, Herning, Denmark. Audience: Researchers. Title: Droner til planteværnsopgaver i Californien. January 17, 2018.
Christian Nansen: Seminar at the Department of Plant and Environmental Sciences, University of Copenhagen, Copenhagen, Denmark. Audience: Researchers. Title: Research into the use of imaging and actuating drones in agriculture. January 11, 2018.
Christian Nansen: Oral presentation at the Annual Santa Maria Strawberry and Vegetable Meeting, Santa Maria, CA, USA. Audience: Strawberry growers, pest control advisers, industry professionals, and researchers. Title: Improving management of arthropod pests in strawberry production systems. November 28, 2017.
Elvira de Lange: Oral presentation at CAPCA ED (California Association of Pest Control Advisers Education), Oxnard, CA, USA. Audience: Pest control advisers and industry professionals. Title: Integrated pest management in California strawberry. June 28, 2017.
Elvira de Lange: Oral presentation at CAPCA ED, San Jose, CA, USA. Audience: Pest control advisers and industry professionals. Title: Integrated pest management in California strawberry. May 3, 2017.
Elvira de Lange: Oral presentation at Entomology 2017, the Annual Meeting of the Entomological Society of America, Denver, CO, USA. Audience: Researchers and industry professionals. Title: Detecting spider mite-induced stress in California strawberry using innovative remote sensing technology. November 5-8, 2017.
Elvira de Lange: Seminar at the Department of Entomology, Rutgers University, NJ, USA. Audience: Researchers. Title: Drone-guided releases of predators and optimized pesticide spray coverage as strategies for sustainable pest management in strawberry. September 22, 2017.
Elvira de Lange: 1st Biotweeps Twitter Conference, online. Audience: Biologists in all disciplines from all over the globe. Title: Drone-guided releases of predators for sustainable pest management in strawberry. June 28-30, 2017.
Elvira de Lange: Seminar at the Department of Population Biology, University of Amsterdam, Amsterdam, Netherlands. Audience: Researchers. Title: Detecting spider mite-induced stress in California crops using innovative remote sensing technology. 24 March 24, 2017.
Attendance only:
Elvira de Lange: Strawberry Breeding Field Day in Ventura County. Audience: Audience: Strawberry growers, pest control advisers, industry professionals, and researchers. April 6, 2018.
Elvira de Lange: 16th Annual Strawberry Production Meeting in Ventura County. Audience: Strawberry growers, pest control advisers, industry professionals, and researchers. September 7, 2017.
Press coverage:
Using drones and predatory bugs, UC Davis researchers treat crop pests. Author: Lauren Glevanik. The California Aggie. January 24, 2020. https://theaggie.org/2020/01/24/using-drones-and-predatory-bugs-uc-davis-researchers-treat-crop-pests/
UC Davis researchers studying the use of drones to identify and mitigate pests in crops. Author: Ryan Hill. CBS13. December 12, 2019. https://gooddaysacramento.cbslocal.com/2019/12/12/uc-davis-researchers-study-drones-identify-pests-crops/
Drones for precision pest control in agriculture. Author: Andy Fell. Egghead (blog about research at UC Davis). December 11, 2019. https://egghead.ucdavis.edu/2019/12/11/drones-for-precision-pest-control-in-agriculture/
UC Davis study finds drones support sustainable farming. Author: Kathy Keatley Garvey. Daily Democrat (Yolo County, CA news). December 10, 2019. https://www.dailydemocrat.com/2019/12/10/uc-davis-study-finds-drones-support-sustainable-farming/
Importance of drones in agricultural practices: UC Davis fellow. Author: Kathy Keatley Garvey. Davis Patch (Davis, CA news). December 9, 2019. https://patch.com/california/davis/importance-drones-agricultural-practices-uc-davis-fellow
Why drones are important in sustainable agriculture in the 21st century. Author: Kathy Keatley Garvey. Bug Squad (blog about happenings in the insect world, University of California). December 9, 2019. https://ucanr.edu/blogs/blogcore/postdetail.cfm?postnum=38935
Elvira de Lange: Drones are increasingly important in sustainable agricultural practices. Author: Kathy Keatley Garvey. Entomology & Nematology News (blog, University of California news about entomology and nematology). December 8, 2019. https://ucanr.edu/blogs/blogcore/postdetail.cfm?postnum=38919
Drone versus spider mite. Author: Eric Butterman. The American Society of Mechanical Engineers. January 19, 2018. https://www.asme.org/topics-resources/content/drone-versus-spider-mite
Drones helping to fight mites in strawberry, almond fields. Author: Liz Kreutz. KXTV, ABC10. June 7, 2017. http://www.abc10.com/news/local/davis/drones-helping-to-fight-mites-in-strawberry-almond-fields/446347500
Researcher uses drones, predators against strawberry pests. Author: Tim Hearden. Capital Press (Agriculture website). June 6, 2017. http://www.capitalpress.com/Research/20170606/researcher-uses-drones-predators-against-strawberry-pest
UC Davis researchers targeting spider mites with bug drone. CBS13. June 1, 2017. http://sacramento.cbslocal.com/2017/06/01/uc-davis-spider-mites/
UC Davis scientist targets spider mites in strawberry fields. Author: Kathy Keatley Garvey. The Davis Enterprise (Yolo County, CA news). May 31, 2017. http://www.davisenterprise.com/local-news/uc-davis-scientist-targets-spider-mites-in-strawberry-fields/
How a UC Davis Scientist is targeting spider mites in strawberry fields. Author: Kathy Keatley Garvey. Davis Patch (Davis, CA news). May 30, 2017. https://patch.com/california/davis/how-uc-davis-scientist-targeting-spider-mites-strawberry-fields
Drones used to fight spider mites in strawberry fields. Author: Kathy Keatley Garvey. Daily Democrat (Yolo County, CA news). May 27, 2017. https://www.dailydemocrat.com/2017/05/27/drones-used-to-fight-spider-mites-in-strawberry-fields/
Innovative research: How Elvira de Lange is targeting spider mites in strawberry fields. Author: Kathy Keatley Garvey. Entomology & Nematology News (blog, University of California news about entomology and nematology). May 24, 2017. http://ucanr.edu/blogs/blogcore/postdetail.cfm?postnum=24192
Education and Outreach Outcomes
- Use of drones in sustainable pest management