Progress report for SW22-933
Project Information
California’s 34 million acres of rangelands support diverse social-ecological resources, including some of the world’s major biodiversity hotspots, watersheds for nearly all of the State’s surface waters, and a >$3B annual livestock industry. Climate change and weather extremes threaten the economic viability and environmental sustainability of these highly valued systems. Rangeland management is also challenged by the characteristic climatic and biophysical diversity variability of these systems—resulting in high spatiotemporal variability in supply of ecosystem services, including forage productivity. If California rangeland managers had access to reliable data across these vast, dynamic landscapes, then they would be able to make cost-effective, climate-informed grazing decisions to sustainably enhance productivity and profitability.
Our interdisciplinary team will build accessible decision-support tools based on high spatiotemporal resolution satellite remote sensing data to provide rangeland managers actionable information directly relevant to rangeland sustainability and ranch-level profitability. The proposed tools focus on tracking forage production—a top grazing management goal—and residual forage dry matter (RDM)—a critical rangeland health indicator for California’s annual grasslands.
Our research makes use of multi-scale, multi-source remote sensing imagery, augmented with on-ranch measurements, and provides more realistic range condition monitoring in near real-time. This effort capitalizes on our newly established, collaborative on-ranch monitoring sites across 32 counties. The field-based measurements will be used to further refine and calibrate an algorithm we previously developed for Landsat based forage production estimations. An automatic workflow will be developed to fuse Sentinel-2 10 m imagery every 5 days with Landsat imagery, augmented with higher resolution NAIP and Planet imagery.
We will also develop an innovative machine learning approach to estimate end-of-season RDM, by integrating improved growing season production time series, preceding weather, and soil properties, with concurrent Landsat/Sentinel imagery. The RDM estimation algorithm will be trained with field measured RDM with data augmentation from unique hyperspectral imagery. To reduce potential adoption barriers, we will take advantage of the Google cloud platform and APIs to create accessible data visualization and decision-support tools.
We will engage ranchers and other rangeland stakeholders throughout the project via our project Advisory Group, on-ranch research, and in-person workshops. Extension education resources will be co-developed with our rangeland partners and will include online multimedia (decision-support apps, videos), fact sheets/policy briefs, newsletters, and open access journal publications. We will collect feedback on effectiveness of workshop content and decision-support tools via focus group discussions and on-site evaluations at each workshop.
Near real-time, scalable remote monitoring and decision-support tools, combined with effective and inclusive education, will help us broadly deliver information critical to rangeland agricultural sustainability. The data-driven tools will complement on-ranch monitoring, help ranchers accurately visualize rangeland health and thus optimize livestock grazing management to promote forage productivity and maintain beneficial RDM levels. Expected near- to mid- term outcomes include stakeholder adoption of decision-support tools and enhanced skills in integrating forage production and RDM monitoring into rangeland decision-making. Potential long-term impacts include enhanced sustainability and resilience of California’s rangelands and ranches through better resource management.
Research Objectives
- Collect and compile ground-truthed data in collaboration with UCCE and FSA to build an extensive field-based geospatial database for California.
- Develop a well-calibrated remote sensing algorithm scalable statewide for consistent forage production estimates in near real-time.
- Develop a locally-optimized RDM estimation tool with multi-sensor imagery.
- Prototype an online interactive app to help stakeholders improve ranch management and risk mitigation decision-making.
Education Objectives
- Assemble an Advisory Group of diverse rangeland stakeholders to provide immediately actionable, on-the-ground user input to developing accessible decision-support tools.
- Engage with ranching, policy, research, and management communities to extend research products and collect feedback on tool usability for rangeland decision-making.
This timeline outlines major milestones and activities for project objectives. In support of Research Objectives 1 and 3, on-ranch monitoring sites have already been identified and installed in collaboration with ranchers and rangeland managers across 32 counties (Fig.1).
2022 |
2023 |
2024 |
2025 |
|||||||||
|
Q2 |
Q3 |
Q4 |
Q1 |
Q2 |
Q3 |
Q4 |
Q1 |
Q2 |
Q3 |
Q4 |
Q1 |
Research Objective 1 |
||||||||||||
Clip and measure forage production |
XXX | XXXXXXX | XXXXXXX | |||||||||
Collect RDM measurements |
XXX | XXX | ||||||||||
QA/QC and build the GIS database |
XXX | XXX | XXX | |||||||||
Research Objective 2 |
||||||||||||
Assemble NAIP imagery/environmental/weather data |
XXXXXXXXXXXXXXXXXXXXXXXXXXXXXXXXXXXXXXXXXX |
|||||||||||
Assemble Sentinel, Landsat and Planet imagery |
XXXXXXXXXXXXXXXXXXXXXXXXXXXXXXXXXXXXXXXXXXXXXX | |||||||||||
Generate herbaceous data layer |
XXX | XXX | ||||||||||
Develop multi-sensor data fusion |
XXXXXXXXXXXX | XXX | XXX | |||||||||
Calibrate/optimize light use efficiency parameterization |
XXXXXXXXXXXX | |||||||||||
Develop and test forage production workflow |
XXXXXXX | |||||||||||
Research Objective 3 |
||||||||||||
Customized ordering of PRISMA imagery |
XXXXXXX | XXXXXXX | ||||||||||
Develop and test PRISMA RDM approach |
XXXXXXX | XXXXXXX | ||||||||||
Develop/test operational RDM workflow |
XXXXXXX | |||||||||||
Research Objective 4 |
||||||||||||
Designing ideas for the API functions and dashboard |
XXX | XXXXXXX | ||||||||||
Prototype and implement forage production API |
XXX | XXXXXXX | ||||||||||
Prototype and implement RDM API |
XXXXXXX | |||||||||||
Prototype and test the decision support modules |
XXXXXXX | |||||||||||
App Roll out to the hosting sites and further refinement |
XXXXXXX | |||||||||||
Education Objective 1 |
||||||||||||
Identify project Advisory Group with producer cooperators and partners |
XXX | |||||||||||
Advisory Group meeting: identify types/scales of information relevant to management triggers |
XXX | |||||||||||
Advisory Group follow-up meetings: progress updates, beta-testing, and feedback |
XXX | XXX | XXX | |||||||||
Education Objective 2 |
||||||||||||
Develop in-person workshops with partners |
XXX | XXX | XXX | |||||||||
Promote workshop events, invite target audiences |
XXXXXXXX | XXXXXXXX | XXX | |||||||||
Finalize evaluations tools (workshop surveys/focus group questions) |
XXX | |||||||||||
Stakeholder decision-support tool workshops |
XXX | XXX | XXX | |||||||||
Analyze workshop evaluation data |
XXXXXXX | XXX | XXXXXXX | |||||||||
Develop online multimedia content: online hub, live-recorded presentations |
XXXXXXXXXXXXXXXXXXXXXXXXXXXXXXXXXXXXXXXXXXXXXX | |||||||||||
Develop educational resources: popular articles, fact sheets/policy briefs, newsletters |
XXXXXXXXXXXXXXXXXXXXXXXXXXXXXXXXXXXXXXXXXXXXXXXXXX | |||||||||||
Develop project reports and journal publications |
XXX | XXX | XXXXXXXXXXXXXXXX |
Cooperators
- - Producer
- - Producer
- - Producer
- - Producer
Research
This project focused on California’s rangelands, where climatologically, ecologically, and topographically diverse landscapes pose the biggest challenge for sustainable ranching (Fig. 1). During Year 1 of the project, we made significant progress to address the four interconnected research objectives (Fig. 2). This laid the foundational work for the development of monitoring growth status, forage production, and RDM, to facilitate adaptive management and decision-making.
Research Objective 1. Build an extensive field-based geospatial database on the aboveground biomass metrics over California’s rangeland, to be used to calibrate and evaluate the remote sensing-based monitoring algorithm.
UCCE and FSA collaborated (FSA funded field supplies and Loss Adjuster labor cost) to identify and establish approximately 40 monitoring sites (700 m2) that will provide a representative distribution of annual rangelands across all sub-ecoregions statewide (Fig. 1). In late spring of 2022, we reconvened our partners, revisited the exclosure site selection, and revised sampling and measurement protocol, based on the experiences during previous field season (September 2011 to March 2022). A total of 42 sites were finalized, representing the diversity of the annual grasslands across all sub-ecoregions statewide (Fig. 1). The exact locations of each sites were recorded by high precision Trimble GPS. To match the footprint size of available moderate to high spatial resolution satellite remote sensing data (~10 to 30 m), each site was situated in a large open grassland (> 45 x 45 m, a.k.a., Macro plots) and includes one large exclosure (14.63 x 14.63 m) to accommodate remote sensing and field clip-plot quantification. A total of 17 county based UCCE farm advisors and 5 regional FSA “Loss Adjusters” collected field data in 2021/22 and 2022/2023.
Forage clippings were done once or twice a month from January to March to capture the rapid growth period, once in April/May to capture peak standing crop, and once from late September or October to capture RDM. Plant functional group composition (percent grass vs forb cover) was recorded at each sampling event (2021/2022 and 2022/2023 seasons); additionally, dominant species were recorded via the dry-weight-rank method at each site at peak standing crop (2022/2023 season). For the 2021/2022 season, clippings were specifically collected within non-grazed exclosures and in adjacent grazed areas. A subset of samples were sorted to separate new growth from old-growth (i.e., RDM) to measure the current year’s forage biomass to match satellite based estimations. This approach will allow us to assess the ability of the remote sensing platform to quantify forage biomass in paired grazed vs non-grazed areas, which can differ in build-up of RDM. For the 2022-2023 season, we continued to clip forage samples within grazing exclosures, field sorting samples with greater than 15% RDM cover.
For each monitoring site, 10-15 samples were clipped during each clipping event at each site. The clipping samples were averaged by date for each site, resulting in a total of 150 site-date data points for further analysis. By following the same protocols for sampling, field data collection, and preprocessing, we built a large geospatial database of forage production with high quality and consistency, which will continue to grow as new clipping data comes in.
Research Objective 2. Develop a well-calibrated remote sensing algorithm scalable statewide for consistent forage production estimates in near real-time.
We will improve upon the remote sensing approach that we developed for forage production estimation using MODIS daily 250-m and Landsat 16-day 30-m satellite observations (Liu et al., 2021). Our prior approach was calibrated with limited exclosure site data. Moreover, it was applied only to herbaceous-dominated rangelands, to avoid the mixed woody-herbaceous pixel issue at 30-m resolution. In order to provide daily estimates for the majority of rangelands without the masking effects from shrubs and trees, higher spatial resolution imagery is needed as a main data stream. Since PlanetScope's open California data policy phased out, we relied on Sentinel 2A&B at 10 m every 5 days (plus Landsat), both publicly-available and free, for operational near real-time forage monitoring (Fig. 2). Our focus will be on all 10 ×10-m pixels without trees or shrubs. A first version of the base mask for herbacious cover at 10m was generated by first combining herbaceous or grasslands pixels from California vegetation map, the European Space Agency (ESA) World Cover, and the National Land Cover Database (NLCD). Only pixels with NDVI lower than 0.3 in mid-August were retained for further analysis since the presence of trees and other perennial vegetation within a 10m pixel would increase the NDVI value compared to senescent grasses. In the next step, we will further refine the layer with the meter scale NAIP aerial imagery, available every other year, and updated with Sentinel summer/fall-time imagery when grass is senescent.
To estimate forage production, we adopted the similar biophysical light use approach, developed from our previous studies (Liu et al., 2019, 2021), and worked on further improvement of accuracy and robustness, especially with higher spatial and temporal resolution of Sentinel 2 satellite imagery than previously used Landsat 30m imagery, and leveraging the substantial field data from the newly established network of the exclosure sites for model training and testing.
We assembled weather data, including incoming solar radiation, temperature, precipitation, were also downloaded from CIMIS and PRISM. We also assembled the topographical indices such as elevation and soil data such as soil organic matter. We have downloaded Sentinel 2A & 2B imagery at 10m to 20m with repeated observations every 5 days from 2022 to May 2023, with a total of 16 tiles covering all 42 field sampling sites. An automatic preprocessing workflow has been developed to filter clouds and shadow for each of the 12 spectral bands.
Light interception and light-use efficiency optimization will be further improved by incorporating the three unique red-edge bands from Sentinel-2, which Landsat does not include. Reflectances in the red-edge spectrum is more sensitive to chlorophyll absorption and thus reduces the saturation issue of the previously widely used NDVI at higher biomass levels (Lin et al., 2019). Moreover, the red-edge-based vegetation indices are more sensitive to the spatio-temporal variations of pigment degradation and therefore serves as a proxy for plant stress related with the light-use efficiency reduction (Zarco- Tejada et al., 2018). During Year 1, we calculated a set of vegetation indices for monitoring phenology and growth and plant stress, including the Normalized Difference Vegetation Index (NDVI), the red-edge bands based NDVI, the Simple Ratio (SR), the red edge bands based SR, the Soil Adjusted Vegetation Index (SAVI), and the Normalized Burn Ratio (NBR). The Savitzky-Golay filter was applied to the NDVI time series to fill the gap and remove the abnormal values; a linear interpolation was then performed to generate the daily time series of NDVI. We will evaluate the relationships of various red-edge related indices with measured production and include the most significant ones for the refined approach.
For each individual 10 m grass pixel, we have already identified the phenological metrics in California annual grasslands such as the start of the growing season (SOS), end of the growing season (EOS), and length of the growing season (LOS). The Sentinel-2 NDVI time series of each pixel was fitted with two sigmoidal curves and the rate of change was then used to identify the SOS and EOS and estimate the growing season length, which was fed into the daily accumulation of forage production.
We started to explore the light use efficiency optimization approach with the available field data and extracted Sentinel-2 data. The baseline model was based on a constant light use efficiency determined by the accumulated absorbed photosynthetically active radiation (APAR) and the field measured forage production before and at the peak production. The improved optimization of LUE factored the impact of environmental stressors. We are in the process of including three Sentinel 2 unique red-edge bands that can indicate the moisture stressors constraining plant growth for LUE optimization.
We will use 70% of the field data, randomly selected, for the optimization process, and use the remaining 30% of the data for validation. Uncertainty will be quantified in terms of annual forage production, peak standing biomass and phenology. This remote sensing algorithm will be further optimized as new field measurements become available. The validated remote sensing tool will be implemented over all of the herbaceous-dominated rangeland areas that are within the Mediterranean climate zone.
Research Objective 3. Develop a locally-optimized RDM estimation tool with multi-sensor imagery.
Recent work on variability and loss in RDM over the summer dry period (Larsen et al., 2020) suggests important implications for using remote sensing estimates of both growing and non-growing season above-ground biomass. To map RDM operationally during the dry season, we will develop an innovative and scalable approach using locally optimized machine learning algorithms. Our approach involves two steps: (1) scaling up the field measured RDM with unique hyperspectral measurements from PRISMA imagery acquired over selected large areas, and (2) wall to wall cost-effective RDM mapping with the calibrated machine earning models to integrate improved growing season production estimates, factors related to decomposition, and coincident Landsat/Sentinel imagery.
During Year 1, we focused on preparing for developing a calibrated remote sensing algorithm to estimate RDM using PRISMA hyperspectral imagery at 30-m resolution. We submitted tasking order to acquire PRISMA imager from August 2022 to to cover our monitoring sites. A total of 10 scenes were acquired at a 30 × 30 km swath. Work is on the way to explore the relationship between cellulose absorption features at ~2100 nm and many other spectral features, such as Cellulose Absorption Index and the traditional broadband indices (NDRI) with field measured RDM. Once more field data is collected this fall, a statistical model will then be built to identify the most important hyperspectral features and indices for RDM estimation across sites and estimate RDM with PRISMA data. The calibrated model will then be applied to all herbaceous-dominated 30-m pixels to map RDM for each PRISMA scene. We will build a separate model to incorporate only those broadband indices available from Landsat and Sentinel for RDM estimation, and evaluate the improvement of PRISMA-based RDM method over existing approaches.
Next, in order to upscale the PRISMA approach for operational RDM estimates across the whole state, we will utilize a machine learning approach to integrate forage production in the growing season (Objective 2) with the fall-season Landsat/Sentinel imagery, preceding weather, solar energy, and topography for a more robust RDM estimation. This will leverage the large RDM datasets generated above from PRISMA as training data. Models will be developed for each individual region to account for potential impacts of regional variations in soils, species and decomposition rate. We will estimate RDM for all grass-dominated Sentinel pixels and assess the accuracy with ground measurements.
Research Objective 4. Prototype an online interactive app to help stakeholders improve ranch management and risk mitigation decision-making.
We brainstormed the designing ideas for the API functions and dashboard. While waiting for the field data to be completed, we also assessed the historical annual forage production maps (2001-2018 from Landsat imagery at 30m) produced from our previous studies to quantify and understand potential trend of year to year forage production variability, an important aspect related to ranch management and risk mitigation decision-making. The coefficient of variation (CV), defined as the ratio of the standard deviation divided by the mean, was used to represent the interannual variability in forage production as well as other vegetation and climatic factors. The 8-year moving window from 2001 to 2018 was applied to reduce the uncertainties caused by the disturbances such as drought. We applied the Mann-Kendall and the Theil-Sen methods to test the significance of and quantify the trends of the variability of forage production, for each 30m pixel, and then summarized over each sub-ecoregion. To further understand the trends of variability in forage production, the random forest model was built using a suit of remote sensing indices, climate indices as well as soil and topographical indices were used as predictors. Around 20,000 samples, of which the minimum distance between the points was set as 300 meters, were randomly selected for model building, due to the extremely large samples at 30m. For the random forest modeling, 70% of the sampling points were used for training models, and the left 30% of the points were used for validation. The Root Mean Square Error (RMSE), Mean Absolute Error (MAE) and Variance Explained by the independent variables were used to quantify the accuracy of the model. The performance of the input variables was evaluated by the percent increase in Mean Square Error (%IncMSE). The partial dependence plot was used to quantify how the trends of variations of forage production vary with each independent variable.
We will be prototyping an easy to use API accessible in both desktop and mobile devices, to enhance data access and interactive visualization of near real-time monitoring results. All of the calibrated algorithms for statewide applications will be implemented with Python API on Google Earth Engine (GEE). This workflow automatically ingests the imagery from Landsat series at 30m every 8 days and Sentinel 2 at 10m every 5 days, weather data, as well as the less frequent NAIP imagery at 0.6m to 1m, and topography all available for free on the GEE platform. The imagery stream will be further augmented with available Planet 3m imagery via Planet and GEE cloud integration tools (via Jin’s Ambassador partnership with Planet). Imagery fusion and routine estimates of growing season forage production will be produced at 10m resolution on the fly and updated every 3 to 5 days. The RDM estimates will be generated every 10 days in late season, using the calibrated ML algorithms to integrate the growth curve, and preceding weather, concurrent multispectral imagery, and topographical attributes.
We will work closely with ranchers, local UCCE advisors, and the Advisory Group to co-produce the interactive app, which will guarantee the ultimate product will serve the specific needs of California rangeland managers. A user-friendly dashboard will be designed to allow users to query, visualize, and summarize forage production and RDM at various scales. Various tabs will also permit users to examine spatial heterogeneity within and across management units, which will aid them in designing optimized livestock management and grazing strategies across the landscape. By combining with our previous historical daily forage production estimates at 30m resolution (Liu et al., 2020), end users can visualize deviations from average production (anomalies) at ranch, region, or state-wide scales, and make adaptive decisions. We will also incorporate the machine learning based analysis and models on site-specific forage production sensitivity to weather (Liu et al., 2023). The RDM monitoring will assist producers and land managers to evaluate how closely they are achieving RDM objectives before the end of the season, and adapt their grazing activities accordingly. Examples include determining how many grazing days they would have if they turned cattle into a pasture mid-summer.
We expect this interactive app will profoundly affect in-season on-ranch decision-making. Having access to near-real-time forage production data during the growing season will allow producers who cannot regularly access lands to make critical decisions regarding stocking rates, pasture rotations, and shipping dates with substantially greater precision and accuracy. Additionally, all producers will benefit from features allowing them to query forage production data by date in order to compare production across months and years. Impact and effectiveness of the interactive app will be regularly evaluated in collaboration with project Advisory Group and via extension education workshops (see Education Plan and Evaluation and Producer Adoption sections).
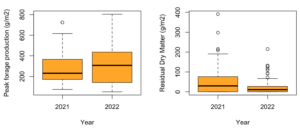
Our initial analysis of the field clipping data showed large variations among sites, with a peak biomass of 285 +/- 174 g/m2 during 2020-2021 and 307 +/- 207 g/m2 during 2021-2022 growing seasons (Figure 3a). The least productive site occurred in Pulis in Tehama county, with a production of 79 g/m2 during 2020-2021 and 51 g/m2 during 2021-2022. In contrast, more productive sites, such as Gordon in Napa county, had a production of 723 g/m2 during the growing season of 2020-2021, and Orvis in Stanislaus county, had the highest forage production of 804 g/m2 during 2021-2022. Much higher production was found in spring 2022 than 2021, most likely due to higher precipitation. Compared to peak biomass, fall RDM measurements showed even larger variability across sites (Figure 3b), 154+/-140 g/m2 fall 2021 vs 228 +/- 212 g/m2 in fall 2022. The lowest 2021 fall RDM also occurred in the Pulis site (7.4) g/m2), while the CPW6 site in San Luis Obispo county had the largest RDM of 394 g/m2.
As a first step, the baseline model optimization with a constant LUE showed that the estimated forage production agreed reasonably well with the field data with a coefficient of determination of 0.74 and RMSE of 53.38 g/m2, based on the 30% of the data used for testing (Figure 4). The further optimization of LUE as a function of environmental stressors with weather and remotely sensed stress indicators from Sentinel 2 will improve the forage production estimation significantly at the next step.
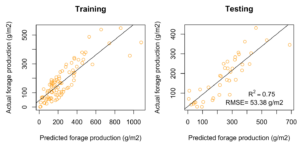
The analysis of the historical forage production maps at 30m from 2001- 2018 showed an increasing trend of year to year fluctuation in forage production over many ecoregions in California annual grasslands. The trends were found significant in many subsections including Ultrabasic Complex, Tehama Terraces, Lower Foothills Metamorphic Belt and Lower Granitic Foothills. The random forest models built explained 83.43% of the variance in the trends of the variability in forage production, when using a combination of climate, topographic and soil variables. The key environmental variables driving the observed fluctuation trend included long-term mean annual precipitation, long-term annual minimum temperature, and soil organic matter. We found that drier areas, especially when mean annual precipitation less than 700 mm/year, were very sensitive to changes in precipitation, and drought would lead to larger interannual variability, making ranching decisions more challenging (Figure 5). Over areas with warmer winter, especially the annual minimum temperature between 7 and 8.5 C, warming would enhance the year to year fluctuation. Regions with higher soil organic matter were found to be more resilient to climate change (Figure 5).
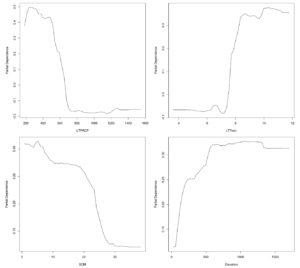
Research Outcomes
Not yet
Education and Outreach
Participation Summary:
To support stakeholder education and ensure project success, we have assembled an interdisciplinary team of scientists and public and private resource managers (see letters of support) to collaboratively identify critical gaps and inform design and development of the proposed decision support tools. All cooperating producers on the project proposal have already been actively involved via the initial on- ranch monitoring (Research Objective 1), as have an additional 35 ranchers across California.
Our producer cooperators are long-time collaborators with UCCE and are committed to engaging in all project stages, including advising on scope of data collection, interpretation of research results, identification of potential barriers for technology adoption, and development of support tools. We will also continue to engage a diversity of stakeholders through a project Advisory Group (Education Objective 1), 3 project workshops (Education Objective 2), and evaluation and assessment of project outputs.
Education Objective 1. Assemble an Advisory Group of diverse rangeland stakeholders to provide immediately actionable, on-the-ground user input to developing accessible decision-support tools.
We will establish a project Advisory Group to consult on design, development, and implementation of the proposed project outputs. We will work with our collaborators and partner networks including producer associations (e.g., California Rangeland Trust, California Cattlemen’s Association); public agencies (e.g., County Agricultural Commissioners, USDA California Climate Hub, USDA Farm Service Agency, USDA Natural Resources Conservation Service); UC Cooperative Extension (UCCE); and environmental non-governmental organizations (e.g., Audubon California) to identify candidate Advisory Group members (see letters of support). The Advisory Group will be comprised of approximately 15 individuals, with ~50% of the group represented by livestock producers, ~30% represented by natural resource managers from local, state, and federal agencies, and ~20% represented by resource managers from environmental non-governmental organizations.
The Advisory Group was identified, invited, and convened by the third quarter of project year 1. Advisory group members were selected to represent a diversity of backgrounds and expertise—including private and public land managers, small and large producers, multi- generation and first generation producers—to ensure project outcomes address a broad range of management, technical, and socioeconomic barriers for all stakeholders. The Advisory Group will provide input on decision-support tool development via annual project team meetings held in Davis, CA or via virtual meetings if necessary. The initial meeting will focus on identifying stakeholder needs in terms of types and scales of information most relevant to critical management triggers. Subsequently, we will convene the Advisory Group annually in conjunction with the Extension workshops (fourth quarter of each year; see Education Objective 2) for follow-up discussions on decision-support tool progress. The members will also have opportunities to provide ongoing, informal feedback through the Extension/Outreach Representative and the Producer Advisor Representative. We will evaluate progress toward our objectives of building accessible, user-friendly support tools via feedback from the Advisory group as well as the Extension workshops (see Evaluation and Producer Adoption section).
Education Objective 2. Engage with ranching, policy, research, and management communities to extend research products and collect feedback on tool usability for rangeland decision-making.
The project team will work with the Advisory Group (see Extension Objective 1) to extend research products and engage with ranching, policy, and management communities through a combination of in-person workshops, online multimedia, and Extension education
In-person workshops. We will host one in-person workshop in each year of the project (three total workshops). We will target livestock producers, natural resource managers and policy decision-makers from public agencies, and resource managers from environmental non- governmental organizations to bring together a diversity of management, policy, technology, and science expertise at the project workshops. By the second quarter of the project, we will work with our partners from California Cattlemen’s Association, California Rangeland Trust, USDA California Climate Hub, and Audubon California (see letters of support) to promote project workshops and identify and invite stakeholders to participate in the decision-support tool development, as well as provide feedback based on their organizational needs. We will also specifically engage a range of producer operations—including large producers as well as small, moderate sized, and socially disadvantaged producers who often have fewer resources and less access to technical assistance (Munden-Dixon et al., 2019; Roche, 2016). This will ensure scalability of project results—providing capabilities for large to small operations as well as operations of varying structure. Project team members have a track record of engaging diverse stakeholders through their research, extension, and education programs. We have worked extensively with rural communities across California to conduct needs assessments and gain detailed understandings of the range of barriers and challenges faced by a diversity of operators and land managers, ranging from multi- generational, conventional producers to first-generation and beginning ranchers (Munden-Dixon et al., 2019; Roche, 2016; Roche et al., 2015).
The first workshop (fourth quarter of year 1) will focus on communicating our initial remote sensing results, plans for application development, and acquiring stakeholder feedback on app user interface and functionality. The second workshop (fourth quarter of year 2) will focus on sharing timely project results and collecting stakeholder input on the user interface and functionality of the developing decision-support tools. For both workshops, we will use a modified “flipped-classroom” approach: participants will have the opportunity to review brief summaries and materials before the workshop; we will then provide a short recap during the workshop and focus more on hands-on activities and discussing potential features that can be integrated from tools available in other regions (e.g., ‘what-if scenarios’ provided by Grass-Cast in the Great Plains). This approach will provide more time to directly engage participants and collect feedback on management decision-making needs and preferences in terms of usability. The third workshop (fourth quarter of year 3) will center around rolling out the online decision-support applications, including providing opportunities for on-site beta-testing and discussion. This capstone workshop will also host research presentations and facilitated discussions on enhancing sustainability and resilience to climate change risks on California’s extensive working rangelands, including managing rangeland productivity and health in the face of extreme drought and wildfire. We will collect participant feedback on effectiveness of workshop content and the decision support tools via small and large group discussions, as well as on-site evaluations at each workshop (see Evaluation and Producer Adoption section).
Online multimedia. To broaden educational outreach, we will provide easy online access to project information and extension education materials. The interactive decision-support tools and relevant resources on best management practices will be integrated into an online education hub hosted by UC Rangelands (https://rangelands.ucdavis.edu/research), as well as the USDA CA Climate Hub’s web-based compendium of tools and data, Cal-Agroclimate
(http://34.222.10.9), to ensure broad access. As an example of potential reach, the UC Rangelands Research and Information Center receives ~2K pageviews/month and has reached 160 countries. Cal-AgroClimate is in the prototype phase and focuses on production agriculture with crop-specific adaptation planning tools for agricultural professionals. The Cal- AgroClimate platform can be expanded to co-host tools for the rangeland sector, enhancing access to a broad range of stakeholders.
Online training materials will include the web-based decision- support applications, short informational videos (e.g., UC Rangelands and UC ANR YouTube channels), and live-recorded presentations (e.g., via Kaltura Capture). The online platforms will allow us to communicate ongoing project progress and results to a wider range of stakeholders, including rangeland and conservation professionals not directly engaged in project development. Online hub and multimedia content development will start by the fourth quarter of year 1 and will be completed by project end. Project updates and resources will also be broadly shared through social media and local UCCE websites and stakeholder e-newsletters
Extension education resources. In addition to the online training materials, educational resources will include popular press articles (e.g., Progressive Cattleman), fact sheets, policy briefs, and local UCCE newsletters. We will also work with industry partners, such as California Rangeland Trust and California Cattlemen’s Association, to provide outreach via legislative bulletins and newsletters. We will develop presentation materials to share at scientific meetings (e.g., Society for Range Management), producer meetings (California Cattlemen’s Association & CattleWomens Inc Convention), and local UCCE meetings. We will start developing these educational resources by the third quarter of year 1 and will continue through to completion of the project.
Lastly, we will develop five open access peer-reviewed journal publications. We will target open access, management-focused, and high impact scientific journals to provide applied, science-based information to resource managers and to reach a broad scientific and policy audience. Publication development will occur throughout the project.
We have been working with our stakeholder advisory group to collect on-ranch field data as well as gather ongoing project feedback via informal conversations and project virtual meetings. We have shared new and emerging results from this participatory research with both ranchers and natural resource managers and technical advisors. We presented this project to livestock producers during the annual California Cattlemen's Association and California CattleWomen Convention (100 rancher attendees; December 2022 poster session) and to a diversity of rangeland managers and scientists at the Society for Range Management Annual Meeting (~200 attendees; February 2023 poster session). These opportunities allowed us to get the project in front of a broad range of ranchers and managers and better understand the questions they have about this type of agricultural technology effort. Additionally, in April 2023 we shared this work during a technical field tour of one of the monitoring sites in central California (50 attendees; 2 ranchers and 48 non-ranchers). The field tour allowed us to discuss the project with agricultural technical service providers and ranching cooperators and gain initial feedback on research questions and practical information needs, as well as approaches to make study results and outreach materials accessible to a broad range of stakeholders. We have also presented this ongoing work to a broad audience via the Working Rangelands Wednesdays webinar (Sept 2022; 34 attendees).
Most recently, we had a unique opportunity to lead a rancher focus group in northern California on climate-smart agriculture in which we shared our initial remote sensing results and plans for decisions-support tool development (Jul 2023; 7 rancher attendees). This focus group allowed us to effectively collect stakeholder feedback on potential application development; we are currently analyzing the focus group data. We are also planning another focus group workshop for October 2023 in northeastern California, where we will share more project results and collect stakeholder input on the developing decision-support tool.
Once the developing decision-support tool is ready for beta-testing, we will develop extension education materials, workshops for beta-testing the tool, and resources for stakeholders.
Education and Outreach Outcomes
No learning outcomes yet