Project Overview
Information Products
Commodities
- Animal Products: dairy
Practices
- Animal Production: animal protection and health
- Education and Training: on-farm/ranch research
Abstract:
Dairy cows are particularly sensitive to heat stress, which can affect profitability due to reduced milk production and reproductive performance, and significantly deteriorate animal welfare. Climate change is likely to increase average temperatures and the frequency of severe heat waves across the US, and recent research suggests that the North Central Region is not an exception to this near-future crisis. The increasing pressure of heat stress demands more active methods of cooling, such as strong ventilation fans and spray water, which use large amounts of electricity and water. The rising cost of electricity and changes in rainfall patterns make it more challenging to maintain the profitability and sustainability of the dairy industry under severe heat stress.
For the optimal mitigation of heat stress, it is crucial to detect the signs of heat stress early before it makes long-term impacts. Traditionally, farmers have used dairy cattle’s individual behavior–how they move, how much they eat and drink, where they spend time, etc.–and herd behavior–how they interact with each other–to indirectly gauge the level of heat stress and take necessary mitigation measures. However, it is becoming less viable as more farms are consolidated and the number of cows managed per farmer rapidly grows.
This research proposes to use computer vision and artificial intelligence (AI) for early detection of heat stress in dairy cattle for more sustainable and profitable dairy farming in the North Central Region. We use state-of-the-art computer vision and AI technologies to monitor and analyze dairy cattle’s individual and herd behavior to help farmers and ranchers make optimal decisions to mitigate heat stress in a timely manner.
This research project introduces a comprehensive approach to precise and personalized cattle monitoring using AI and computer vision for early heat stress prediction.
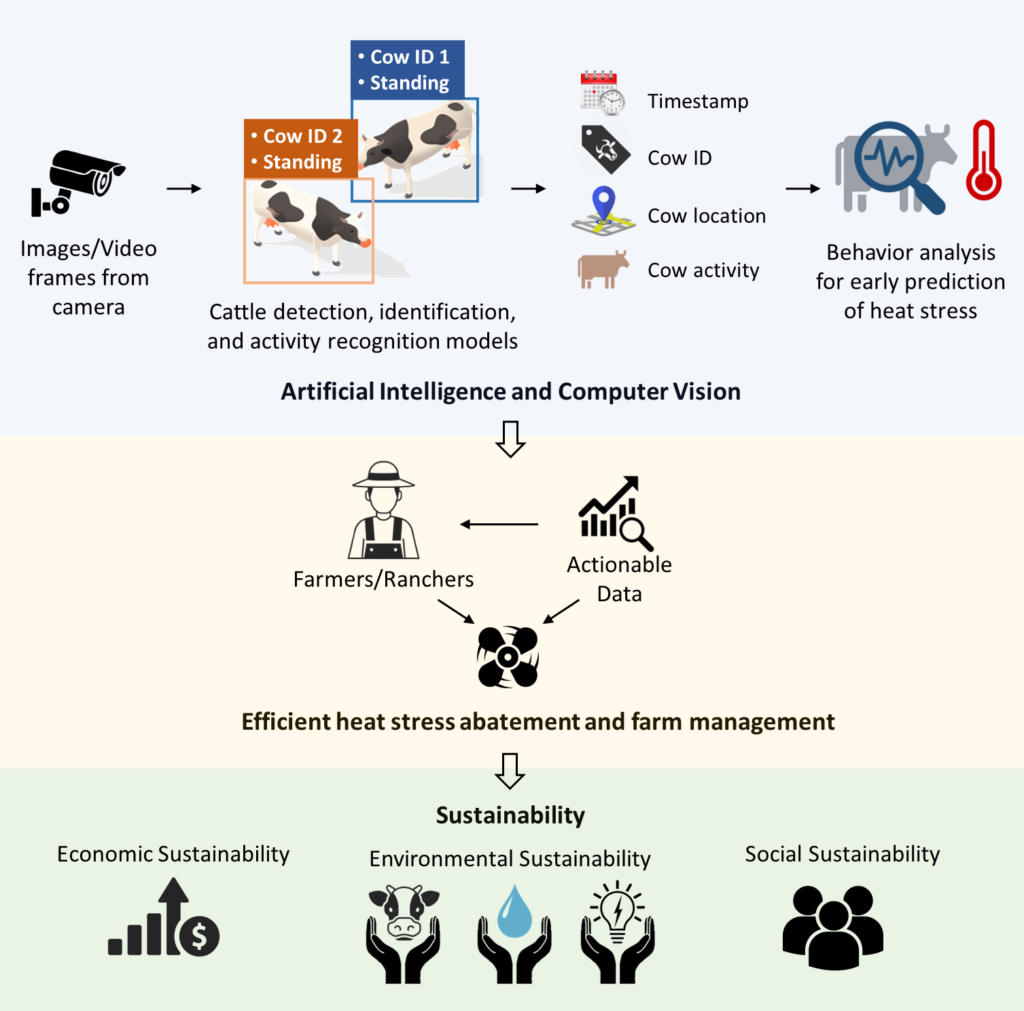
Figure 1 illustrates the proposed methodology. Images/video frames from the cameras installed inside the barn are fed into a computer vision system. It comprises of machine learning (ML) models for cattle detection, individual identification, and activity recognition. The output generated from our computer vision system serves as a crucial source of information, offering valuable indicators closely linked to the occurrence of heat stress in dairy cattle. This personalized and precise data empowers farmers with actionable insights, enabling them to make well-informed and data-driven decisions in a timely manner. This promotes sustainable livestock management and animal welfare.
The outcomes of the proposed research will be disseminated to local farmers through our outreach and extension network. For more wide-spread adoption, we will open-source the software outcomes of the research, including collected and labeled datasets, trained computer vision models, source code, and documentation for training and inference.
Learning outcomes:
Farmers/ranchers will learn how AI can be leveraged for early prediction of heat stress in dairy cattle. They will learn about the changes in behavior in cattle which can be early indicators of heat stress and how automation in the task of behavior analysis using AI can be used in heat stress prediction. It will make them knowledgeable in performing data-driven decision-making to maximize profitability with minimal resources. The project will spread awareness among farmers and ranchers about reducing energy and water consumption through precision livestock and efficient cooling mechanisms. They will learn how they can play a central role in reducing energy and water footprint of a barn leading to sustainable livestock practices.
Researchers can use the dataset collected and labeled as a part of this project and the proposed methodologies to advance their research in the domains of heat stress mitigation, animal welfare, and sustainable agriculture using AI.
Action outcomes:
Farmers/ranchers will use the outputs of the project to mitigate financial losses incurred due to heat stress in cattle using efficient cooling techniques, for continuous monitoring of activities and location of the cattle, and to improve animal welfare. Apart from the direct utility for farmers/ranchers, the output of the project can also be used by engineers and researchers to design energy-efficient, optimal, and cost-effective cooling mechanisms to mitigate heat stress in dairy cattle. Eventually, the outputs of this project will also encourage farmers to move toward more energy-efficient and precise heat stress abatement techniques, contributing to sustainable livestock practices.
The results obtained from our methodology demonstrate its effectiveness in cattle monitoring within a barn environment. The robust performance in cattle detection, individual cow identification, and activity recognition attest to the method's practical applicability in enhancing animal welfare and farm management. Accurate cattle detection forms the foundation for subsequent monitoring phases, while individual cow identification enables personalized monitoring. The integration of these components culminates in a comprehensive understanding of cattle behavior and activity, contributing to improved decision-making. Furthermore, the integration of data from multiple cameras within the multi-camera setup allows for a broader field of view, enhancing the precision and comprehensiveness of cattle monitoring. The results and insights obtained from our methodology demonstrate a significant step towards the development of an advanced cattle monitoring system. It holds promise for a range of applications, from early heat stress prediction to optimizing farm management practices. The robustness of our approach, as evidenced by the quantitative results, underscores its potential to enhance animal welfare, increase farm productivity, and contribute to the overall sustainability of dairy farming operations.
Project objectives:
The primary objectives of this project are as follows:
-
-
Cattle Detection: In order to perform behavior recognition and prediction of heat stress, it is imperative to first detect the individual cows in an image or video frame. We employ the YOLOv7 (You Only Look Once) object detection framework to accurately locate and track cattle within the barn. It is a state-of-the-art real-time object detector in terms of both speed and accuracy, making it a suitable choice for monitoring livestock.
-
Individual Identification of cows: This objective of individual cow identification provides not only accurate detection of cattle but also the ability to differentiate individual cows within the herd, enabling personalized monitoring of the cows. Our approach includes a deep learning-based classifier that is capable of recognizing individual cattle within the barn. This is essential for tracking the behavior and health of each cow, facilitating personalized care and monitoring.
-
Activity Classification: Understanding the activity of cattle is crucial for assessing their health and well-being. The utilization of the activity recognition model not only enhances our understanding of individual cattle behavior but also provides valuable insights into the collective dynamics of the herd. We employ a convolutional neural network-based classifier to distinguish between two key activities: "standing" and "lying." This classification provides insights into the behavior and comfort of the cattle.
-
Generation of a Comprehensive 2D Barn Map: This map serves as a valuable tool for farm management, allowing for the efficient monitoring of each cow's location, identification, and activity status. By applying a linear perspective transformation, we convert the isometric view of the barn into a top-down view, enabling the display of cattle positions, individual IDs, and activity status on the map.
-
Data collection: Good quality data is the key enabler of modern AI algorithms. One of the important research objectives of this project is to collect and label a high-quality labeled dataset for the tasks of cattle detection, identification, and heat stress prediction.
-